Solving Doubly Bordered Tridiagonal Linear Systems via Partition ()
1. Introduction
Linear systems are among the most important and common problems encountered in scientific computing. The whole range of technical problems leads to the solution of systems of linear equations. The first step in numerical solution of many problems is a choice of an appropriate algorithm.
Throughout this paper the word, “simplify”, means simplify the expression under consideration to its simplest rational form. The abbreviation DBT refers to doubly bordered tridiagonal.
The main objective of the current paper is to construct efficient algorithms for solving DBT linear system of the form:
(1)
where the coefficient matrix A is a DBT matrix with the special structure of the partitioned form
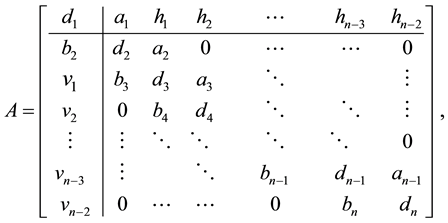
and,.
Such systems arise in many scientific and engineering applications when considering certain partial differential equations and spline approximation. For more details see, for instance [1] -[5] . It is worth noting that in [6] , the authors solved the system (1) via transformation method. In this paper, we are going to solve the system under consideration via partition.
The linear system (1) can also be written in block form as follows:
(3)
where
(4)
is a
block matrix,
, 
, and is
tridiagonal matrix which is given by
(5)
The present paper is organized as follows. In Section 2, we introduce some facts about tridiagonal matrices and a new recursive procedure for inverting this matrix is proposed. In Section 3, the UL factorization of DBT matrix is considered. Numeric and symbolic algorithms for evaluating DBT matrix determinant are constructed. Also a symbolic algorithm for computing the inverse of DBT matrix is developed using the Sherman-Morrison- Woodbury formula. Finally, the solution of the linear system whose coefficient matrix is of DBT matrix type is proposed. In Section 4, some illustrative examples are given.
2. Preliminaries and Basic Results
Tridiagonal matrices arise in many contexts in pure and applied mathematics. Also, they arise in many different theoretical fields, especially in applicative fields such as spline approximation, numerical analysis, ordinary and partial differential equations, solution of linear systems of equations, engineering, telecommunication system analysis, system identification, signal processing (e.g., speech decoding), partial differential equations and naturally linear algebra. See [1] - [4] . Research area on these types of matrices is very active and has recently attracted the attention of many researchers.
In many of these areas, inversions of tridiagonal matrices are necessary and different approaches are considered [7] - [10] in order to obtain such inverse. Some authors consider a general tridiagonal matrix of finite order and then describe the LU factorizations, determine the determinant and inverse of a tridiagonal matrix under certain conditions [1] , [2] and sometimes without any restrictive conditions [8] , [11] . The interested reader may refer to [12] - [23] .
The general tridiagonal matrix
takes the form
(6)
in which
for
.
When we consider the matrix T defined by (6), it is helpful to introduce the n quantities
as follows:
(7)
Following [8] , we have the following result whose proof will be omitted for the sake of space requirement.
Theorem 2.1. The UL factorization of the matrix T in (6) is possible if
for each
. In this case we have the following two UL factorizations:
(8)
where
(9)
and
(10)
The following are two useful remarks:
Remark 1: The matrices
in (9) and (10) are related by
(11)
and
(12)
where
(13)
Remark 2: If the UL factorization of the matrix T in (6) is possible, then we have:
(14)
where
are given by (7). In other words, the matrix T is invertible as long as
(15)
Now we are going to state the following result without proof.
Theorem 2.2. Let T be a non-singular tridiagonal matrix, given by (6), and let
then for
we have
(16)
where ![]()
3. Doubly Bordered Triangular Matrix (DBT Matrix)
In this section, we are going to construct new numeric and symbolic algorithms to evaluate the determinant of a DBT matrix and to compute the inverse of such matrices. For these purposes it is advantageous to consider the UL factorization.
To factor the n × n DBT matrix A given in (2) into the product of an upper-triangular matrix
and a lower-triangular matrix
in the form A = UL, we may proceed as follows
(17)
where
(18)
and
(19)
Equation (17) can be written in block matrix form as follows:
(20)
where
,
and
are the lower and upper triangular matrices of the tridiagonal matrix
given in (5), respectively.
From (20), we see that the following equations are satisfied:
(21)
(22)
(23)
and
(24)
Solving (21), (22) and (23) for
,
and
yields:
(25)
(26)
and
(27)
where
(28)
3.1. Algorithms for Evaluating the Determinant of a DBT Matrix
The determinant of the matrix A in (2) can be computed using the following numeric algorithm.
Algorithm 3.1. A numeric algorithm for computing the determinant of a DBT matrix.
To compute the determinant of the DBT matrix in (2), we may proceed as follows:
INPUT: Order of the matrix, n and vectors a, d, b, h, v.
OUTPUT: The determinant of the matrix A in (2).
Step 1: Set
.
Step 2: For i = n − 1, n − 2, …, 2 do
compute and simplify ![]()
End do.
Step 3: Compute e1 using (27).
Step 4: ![]()
As can be easily seen, Algorithm (3.1) breaks down if any ei = 0 for some
. The following symbolic algorithm is developed in order to remove the case where the numeric algorithm fails.
Algorithm 3.2. A symbolic algorithm for computing the determinant of DBT matrix.
To compute the determinant of the DBT matrix in (2), we may proceed as follows:
INPUT: Order of the matrix, n and vectors a, d, b, h, v.
OUTPUT: The determinant of the matrix A in (2)
Step 1: Set
. If
then
End if. (t is just a symbolic name)
Step 2: For i = n − 1, n − 2, …, 2 do
compute and simplify:
If
then
End if.
End do.
Step 3: Compute and simplify e1 using (27).
Step 4: Compute and simplify:
![]()
Step 5: Det (A) = p(0).
The Algorithm (3.2) will be referred to as DETGDBTRI algorithm.
3.2. Algorithm for Inverting DBT Matrix
In this sub-section, we are going to formulate a new symbolic algorithm for inverting the general DBT matrix based on the Sherman-Morrison-Woodbury formula.
A general DBT matrix can be written as following:
(29)
Applying the Sherman-Morrison-Woodbury formula [13] to A gives
(30)
Now we are ready to formulate the following symbolic algorithm for inverting the DBT matrix.
Algorithm 3.3. A symbolic algorithm to compute the inverse of a non-singular DBT matrix.
To invert a general DBT matrix (2), we may proceed as follows:
INPUT: The order of the matrix, n and the entries of the matrices T, U and V.
OUTPUT: The inverse of the DBT matrix A given in (2).
Step 1: Set
. If
then
. (t is just a symbolic name)
Step 2: For
do
compute and simplify:
. If
then
End if
End do.
Step 3: Compute and simplify the elements of the inverse matrix
using (16).
Step 4: compute
using (30).
Step 5: Substitute t = 0 in all expressions of the matrix
.
3.3. Solving Linear System of Equations of DBT Matrix Type
In this sub-section, we introduce different approaches for solving doubly bordered tridiagonal linear systems of the form (1).
The inverse matrix of the DBT matrix A introduced in (2) could be written in the block form as follows:
(31)
where
,
and![]()
Now, we can formulate our first algorithm for solving the linear systems of the form (1).
Algorithm 3.4. A first numeric algorithm for solving linear systems of DBT matrix type.
To solve a general bordered tridiagonal linear system of the form (1), we may proceed as follows:
INPUT: The entries of the matrix
, the value of d1 and the vectors
.
OUTPUT: The solution vector
.
Step 1: Compute
.
Step 2: Compute
.
Step 3: The solution vector is
.
As we can see, the computational cost of Algorithm (3.4) is
.
The following algorithm depends on the UL factorization of the coefficient matrix A in (1), so it is more convenient to rewrite the linear system (1) as:
(32)
To solve the linear system (32), it is equivalent to solve the two standard linear systems:
(33)
and
(34)
where
and
.
Armed with the above results, we may formulate the following numeric algorithm:
Algorithm 3.5. A second numeric algorithm for solving linear systems of DBT matrix type.
To solve a general doubly bordered tridiagonal linear system of the form (1), we may proceed as follows:
INPUT: The components,
and
.
OUTPUT: The solution vector
of the linear system in (1).
Step1: Use the algorithm (3.1) to check the non-singularity of the coefficient matrix A of the system (1).
Step2: If Det (A) = 0; then OUTPUT (‘no inverse exists’); STOP.
Step 3: Set
.
Step 4: For
do
compute ![]()
End do.
Step 5: Compute ![]()
Step 6: Set
.
Step 7: Compute ![]()
For
do
compute ![]()
End do.
Step 8: The solution vector ![]()
To remove all cases in which Algorithm (3.5) fails, it is convenient to give the following symbolic version of the numeric algorithm described above.
Algorithm 3.6. A symbolic algorithm for solving linear systems of DBT matrix type.
To solve a general doubly bordered tridiagonal linear system of the form (1), we may proceed as follows:
INPUT: The components,
and
.
OUTPUT: The solution vector
of the linear system in (1).
Step 1: Use the DETGDBTRI algorithm to compute
.
Step 2: Use the DETGDBTRI algorithm to check the non-singularity of the coefficient matrix A of the system (1).
Step 3: If Det(A) = 0; then OUTPUT (‘no inverse exists’); STOP.
Step 4: Compute and simplify
using (25).
Step 5: Compute and simplify
using (26).
Step 6: Set
.
Step 7: Compute and simplify
using (28).
Step 8: For
do
Compute and simplify:
![]()
End do
Step 9: Compute and simplify:
![]()
Step 10: Set
.
Step 11: Compute and simplify:
![]()
For
do
Compute and simplify:
.
End do.
Step 12: Substitute t = 0 in all expressions of the solution vector ![]()
The computational cost of Algorithm (3.6) is
multiplications/divisions and
additions/ subtractions. The Algorithm (3.6) will be referred to as DBTLSys algorithm.
4. Illustrative Examples
In this section we give four examples for the sake of illustration.
Example 4.1. Solve the following periodic tridiagonal linear system of size 12.
(35)
Solution: we will solve this system as a DBT linear system where
![]()
By applying DBTLSys algorithm, we have
・ ![]()
・ ![]()
・ The solution vector is
.
This result is in complete agreement with the result in [14] .
Example 4.2. Solve the following DBT linear system
(36)
Solution: we have a DBT linear system with
![]()
By using DBTLSys algorithm we have
・ ![]()
![]()
・ The solution vector is
.
This result is in complete agreement with the result in [6] .
Example 4.3. Solve the following DBT linear system
(37)
Solution: we have a DBT linear system with
![]()
By using DBTLSys algorithm we have
・ ![]()
・ ![]()
・ The solution vector is
.
Example 4.4. Solve the following DBT linear system
(38)
Solution: we have a DBT linear system with
![]()
By using DBTLSys algorithm we have
・ ![]()
・ ![]()
・ The solution vector is ![]()
NOTES
*Corresponding author.