Estimation of Generalized Pareto under an Adaptive Type-II Progressive Censoring ()
1. Introduction
In life testing and reliability studies, the experimenter may not always obtain complete information on failure times for all experimental units. Data obtained from such experiments are called censored data. Reducing the total test time and the associated cost is one of the major reasons for censoring. A censoring scheme, which can balance between, total time spent for the experiment, number of units used in the experiment and the efficiency of statistical inference based on the results of the experiment, is desirable. The most common censoring schemes are Type-I (time) censoring, where the life testing experiment will be terminated at a prescribed time T, and TypeII (failure) censoring, where the life testing experiment will be terminated upon the r-th (r is pre-fixed) failure. However, the conventional Type-I and Type-II censoring schemes do not have the flexibility of allowing removal of units at points other than the terminal point of the experiment. Because of this lack of flexibility, a more general censoring scheme called progressive Type-II right censoring has been introduced. Briefly, it can be described as follows: Consider an experiment in which n units are placed on a life testing experiment. At the time of the first failure,
units are randomly removed from the remaining
surviving units. Similarly, at the time of the second failure,
units from the remaining
units are randomly removed. The test continues until the
-th failure at which time, all the remaining
units are removed. The
are fixed prior to the study. We note that prior to the experiment in the progressive Type-II right censoring, an integer
is determined and the progressive Type-II censoring scheme
with
and
is specified. During the experiment, the i-th failure is observed and immediately after the failure, Ri functioning items are randomly removed from the test. We denote the m completely observed (ordered) lifetimes by
, which are the observed progressively Type-II right censored sample. For convenience, we will suppress the censoring scheme in the notation of the
’s. We also denote the observed values of such a progressively Type-II right censored sample by
Readers may refer to Balakrishnan [2] and Balakrishnan and Aggarwala [3] for extensive reviews of the literature on progressive censoring.
Recently, Ng, et al. [1] suggested an adaptive Type-II progressive censoring, where we allow
to depend on the failure times so that the effective sample size is always m, which is fixed in advance. A properly planned adaptive progressively censored life testing experiment can save both the total test time and the cost induced by failure of the units and increase the efficiency of statistical analysis.
Arandom variable X is said to have generalized Pareto (GP) distribution, if its probability density function (pdf) is given by
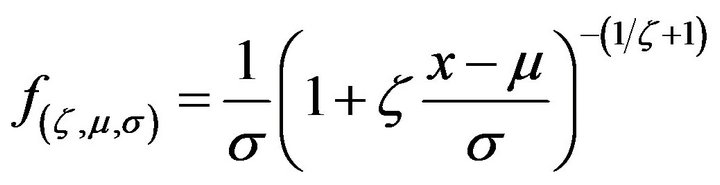
where
and
. For convenience, we reparametrized this distribution by defining
and
. Therefore,
(1)
The cumulative distribution function (cdf) is defined by
(2)
Here
and
are the shape and scale parameters, respectively. It is also well known that this distribution has decreasing failure rate property. This distribution is also known as Pareto distribution of type II or Lomax distribution. This distribution has been shown to be useful for modeling and analizing the life time data in medical and biological sciences, engineering, etc. So, it has been received the greatest attention from theoretical and applied statisticians primarily due to its use in reliability and lifetesting studies. Many statistical methodes have been developed for this distribution, for a review of Pareto distribution of type II or Lomax distribution see Lomax[4], Habibullh and Ahsanullah [5], Upadhyay and Peshwani [6] and Abd Ellah [7,8] and rewferences of them. Agreat deal of research has been done on estimating the parameters of a Lomax using both classical and Bayesian techniques.
The rest of this paper is organized as follows. In section 2, we describe the formulation of an adaptive type-II progressive censoring scheme as described by Ng, et al. [1]. The MLEs of the parameters
and
, approximate confidence intervals are presented in Section 3. Bootstrap confidence intervals presented in Section 4. We cover Bayes estimates and construction of credible intervals using the MCMC techniques in Section 5. Numerical examples are presented in Section 6 for illustration. In Section 7 we provide some simulation results in order to give an assessment of the performance of the different estimation method. Finally we conclude the paper in Section 8.
2. An Adaptive Type-II Progressive Scheme
In this section, a mixture of type-I censoring and Type-II progressive censoring schemes, called an adaptive TypeII progressive censoring scheme is discussed. One can refer to Ng, et al. [1]. This method is also used by Cramer and Iliopoulos [9].
Suppose the experimenter provides a time T, which is an ideal total test time, but we may allow the experiment to run over time T. If the m-th progressively censored observed failure occurs before time T (i.e.
), the experiment stops at the time
(see Figure 1). Otherwise, once the experimental time passes time T but the number of observed failures has not reached m, we would want to terminate the experiment as soon as possible.
This setting can be viewed as a design in which we are assured of getting m observed failure times for efficiency of statistical inference and at the same time the total test time will not be too far away from the ideal time T. From the basic properties of order statistics (see, for example, David and Nagaraja [10]), we know that the fewer operating items are withdrawn (i.e., the larger the number of items on the test), the smaller the expected total test time (Ng and Chan [11]). Therefore, if we want to terminate the experiment as soon as possible for fixed value of m, then we should leave as many surviving items on the test as possible. Suppose J is the number of failures observed before time T, i.e.

where
and
. According to the above result on stochastic ordering of first order statistics

Figure 1. Experiment terminates before time T (i.e. xm:m:n < T).
from different sample sizes, after the experiment passed time T, we set
and
. This formulation leads us to terminate the experiment as soon as possible if the
-th failure time is greater than T for
. Figure 2 gives the schematic representation of this situation. The value of T plays an important role in the determination of the values of
and also as a compromise between a shorter experimental time and a higher chance to observe extreme failures. One extreme case is when
, which means time is not the main consideration for the experimenter, then we will have a usual progressive Type-II censoring scheme with the pre-fixed progressive censoring scheme
. Another extreme case can occur when
, which means we always want to end the experiment as soon as possible, then we will have
and
which results in the conventional Type-II censoring scheme.
If the failure times of the n items originally on the test are from a continuous population with cdf
and pdf
for
the likelihood function is given by (see Ng et al. [1])
(3)
where
(4)
3. Maximum Likelihood Estimation
Let
be an adaptive typeII progressive censored order statistics from generalized Pareto (GP) distribution, with censoring scheme
. From (1)-(3), the likelihood function is given by
(5)
where
is defined in (4) and
is used instead of
.
The log-likelihood function may then be written as
(6)
Upon differentiating (6) with respect to
and
, and equating each result to zero, we get the likelihood equations as
(7)
and
(8)
hence from (7) we obtain the ML estimate of
as
(9)

Figure 2. Experiment terminates after time T (i.e. xm:m:n ≥ T).
By using (9) in (8) we obtain
(10)
Since Equation (10) cannot be solved analytically for
, some numerical methods such as Newton’s method must be employed to solve (10) and get the MLE 
3.1. Approximate Interval Estimation
From the log-likelihood function in (6), we have
(11)
(12)
(13)
The Fisher information matrix
is then obtained by taking expectation of minus Equation (11)-(13). Under some mild regularity conditions,
is approximately bivariately normal with mean
and covariance matrix
. In practice, we usually estimate
by
. A simpler and equally valued procedure is to use the approximation

where
is observed information matrix

approximate confidence intervals for
and
can be found by to be bivariately normal distributed with mean
and covariance matrix
. Thus, the
approximate confidence intervals for
and
are
(14)
respectively, where
and
are the elements on the main diagonal of the covariance matrix
and
is the percentile of the standard normal distribution with right-tail probability
.
4. Bootstrap Confidence Intervals
In this section, we propose to use confidence intervals based on the parametric percentile bootstrap method (Boot-p) based on the idea of Efron [12]. The algorithms for estimating the confidence intervals of the parameters using (Boot-p) method is illustrated as the following1) From the original data
compute the ML estimates of the parameters:
and
from Equation (9) and solving the nonlinear Equation (10), respectively.
2) Use
and
to generate a bootstrap sample
with the same values of
,
;
using algorithm presented in Ng et al. [1].
3) As in Step 1, based on
compute the bootstrap sample estimates of
say
.
4) Repeat Steps 2 - 3 N times representing N bootstrap MLE’s of
based on N bootstrap samples.
5) Arrange all
,
, in an ascending order to obtain the bootstrap sample
Where
Let
be the cumulative distribution function of
. Define
for given
The approximate bootstrap
confidence interval of
is given by
(15)
5. Bayes Estimation and Credible Intervals
In this section we describe how to obtain the Bayes estimates and the corresponding credible intervals of parameters
and
when both are unknown. For computing the Bayes estimates, we assume mainly a squared error loss (SEL) function only; however, any other loss function can be easily incorporated.
In some situations where we do not have sufficient prior information, we can use non-informative prior distribution. This is particularly true for our study. For example, the non-informative uniform prior distribution can be used for parameters
and
. The joint posterior density will then be in proportion to the likelihood function.
Here we consider the more important case when
is the shape parameter and
is the scale parameter has independent gamma priors with the pdfs
(16)
and
(17)
Multiplying
by
we obtain the joint prior density of
and
; given by
(18)
Based on the likelihood function of the observed sample is same as (5) and the joint prior in (18), the joint posterior density of
and
given the data is
(19)
Therefore, the Bayes estimate of any function of
and
say
, under squared error loss function is
(20)
It is not possible to compute (20) analytically even when
is known explicitly. Therefore, we propose the approaches of MCMC technique to approximate (20). See, for example, Robert and Casella [13] and Recently, Rezaei, et al. [14].
The MCMC method provides an alternative method for parameter estimation. It is more flexible when compared with the traditional methods. Moreover, probability intervals are available. The probability intervals provide us a reasonable interval estimate about the unknown parameter. In the following subsection, we propose using the MCMC technique to compute Bayes estimates of the unknown parameters and to construct the corresponding credible intervals.
5.1. The Metropolis-Hastings—Within-Gibbs Sampling
The Metropolis-Hastings algorithm is a very general MCMC method first developed by Metropolis, et al. [15] and later extended by Hastings [16]. It can be used to obtain random samples from any arbitrarily complicated target distribution of any dimension that is known up to a normalizing constant. In fact, Gibbs Sampler is a special case of a Monte Carlo Markov chain algorithm. It generates a sequence of samples from the full conditional probability distributions of two or more random variables. Gibbs sampling requires decomposing the joint posterior distribution into full conditional distributions for each parameter and then sampling from them. We propose using the Gibbs sampling procedure to generate a sample from the posterior density function
and in turn compute the Bayes estimates and also construct the corresponding credible intervals based on the generated posterior sample see Soliman, et al. [17,18]. In order to use the method of MCMC for estimating the parameters of the Lomax distribution, namely,
and
. Let us consider independent priors (16) and (17), respectively, for the parameters
and
. The joint posterior density function can be obtained up to proportionality by multiplying the likelihood with the prior and this can be written as
(21)
The posterior is obviously complicated and no closed form inferences appear possible. We, therefore, propose to consider MCMC methods, namely the Gibbs sampler, to simulate samples from the posterior so that samplebased inferences can be easily drawn. From (21), the posterior density function of
given
is proportional to
(22)
It can be seen that Equation (22) is a gamma density with shape parameter
and scale parameter

and, therefore, samples of
can be easily generated using any gamma generating routine. Similarly, the posterior density function of
given
is proportional to
(23)
The posterior density function of
given
Equation (23) cannot be reduced analytically to well known distributions and therefore it is not possible to sample directly by standard methods, but the plot of it shows that it is similar to normal distribution. So, to generate random numbers from this distribution, we use the Metropolis-Hastings method with normal proposal distribution.
Now, we propose the following scheme to generate
and
from the posterior density functions and in turn obtain the Bayes estimates and the corresponding credible intervals.
1) Start with an 
2) Set
.
3) Generate
from Gamma

4) Using Metropolis-Hastings (see, Metropolis et al. [15]), generate
from
with the
proposal distribution. Where
is variances-covariance’s matrix.
5) Compute
,
.
6) Set 
7) Repeat steps 3-6
times.
8) Obtain the Bayes estimates of
and
with respect to the SEL function as

where M is burn-in.
9) To compute the credible intervals of
and
, order
and
as
and
Then the
symmetric credible intervals of
and
become

6. Illustrative Examples
To illustrate the inferential procedures developed in the preceding sections, we choose the real data set which was also used in Lawless (1982-pp 185). These data are from Nelson [19] concerning the data on time to breakdown of an insulating fluid between electrodes at a voltage of 34 k.v. (minutes). The 19 times to breakdown are
0.96 |
4.15 |
0.19 |
0.78 |
8.01 |
31.75 |
7.35 |
6.50 |
8.27 |
33.91 |
32.52 |
3.16 |
4.85 |
2.78 |
4.67 |
1.31 |
12.06 |
36.71 |
72.89 |
|
Example 1. In this case we take
and
. Thus, the adaptive progressive censored sample is 
Example 2. Now consider that
and
and
’s are same as before. In this case the adaptive progressive censored sample is 
The point estimates of the parameters using the maximum likelihood (ML) method and Bootstrap (Boot-p) are presented in Table 1. Because we have no prior information about the unknown parameters, we assume the non-informative prior (prior 0: the joint posterior distribution of unknown parameters is proportional to the likelihood function). Based on the MCMC samples of size 10000 with 1000 as burn-in, the Bayes estimates of
and
are presented in Table 1. Also the 95% asymptotic, Boot-P confidence intervals and also the 95% credible intervals of
and
in Table 2. From these tables as expected the Bayes estimates under the non-informative prior and the MLE are quite close to each other. A trace plot is a plot of the iteration number against the value of the draw of the parameter at each iteration. Figure 3 displays 10000 chain values for the two parameters
and their histograms are shown in Figure 4 with these settings.
7. Monte Carlo Simulations
In order to compare the different estimators of the parameters, we simulated 1000 an adaptive Type-II progressive samples from Lomax distribution with the values of parameters
and different censoring schemes
. The samples were simu

Table 1. The point estimates of MLE, Boot-p and MCMC of α and β.

Table 2. 95% asymptotic, Boot-p and MCMC confidence (credible) intervals of α and β.
lated by using the algorithm described in Ng, et al. [3]. We mainly compare the performances of ML and Bayes estimates with respect to the squared error loss function in terms of mean squared errors (MSEs). We also compare different confidence intervals, namely the confidence intervals obtained by using asymptotic distributions of the MLEs, bootstrap confidence intervals and the symmetric credible intervals in terms of the coverage percentages. All of the computations were performed by (mathematics 7.0) using a Pentium IV processor. To find the Bayes MCMC estimates, we used the noninformative gamma priors for the two parameters (we call it prior 0). Non-informative prior
provides prior distributions which are not proper, we also used an informative priors, including prior 1, a = 1, b = 2, c = 1, d = 2, with the values of previous parameters. We computed the Bayes estimates and
probability intervals based on 10000 MCMC samples and discard the first 1000 values as burn-in. We report the mean squared errors (MSEs) and the coverage percentage (C.V) based on 1000 replications in Tables 3-6.
8. Conclusions
Recently Ng, et al. [3] suggest an adaptive Type-II progressive censoring. A properly planned adaptive progressively censored life testing experiment can save both the total test time and the cost induced by failure of the units and increase the efficiency of statistical analysis. In this article, we have considered the maximum likelihood (ML)and Bayes estimates for the parameters of the generalized Pareto (GP) distribution using adaptive Type-II progressive censoring scheme. Also, we develop different confidence intervals, namely the confidence intervals obtained by using asymptotic distributions of the MLEs, bootstrap confidence intervals and the symmetric credible intervals for the parameters of the generalized Pareto (GP) distribution. A simulation study was conducted to examine and compare the performance of the proposed methods for different sample sizes, and different censoring schemes.
From the results obtained in Tables 3-6, it can be seen that the performance of the MLEs is quite close to that of the Bayes estimators with respect to the noninformative priors, as expected. Thus, if we have no prior information on the unknown parameters, then it is always better to
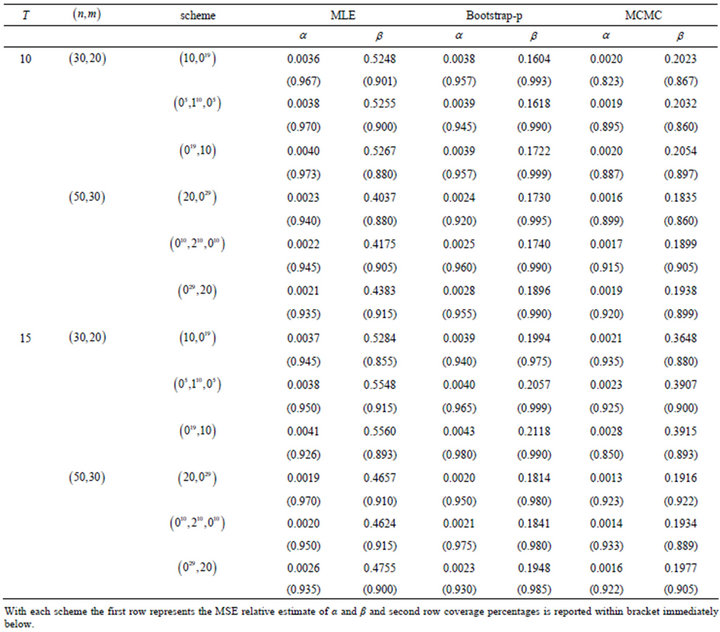
Table 3. Mean squared errors (MSEs) relative estimate of parameters and coverage percentages (C.V) with
and prior 0.

Table 4. Mean squared errors (MSEs) relative estimate of parameters and coverage percentages (C.V) with
and prior 1.

Table 5. The average confidence lengths relative estimate of parameters with
and prior 0.
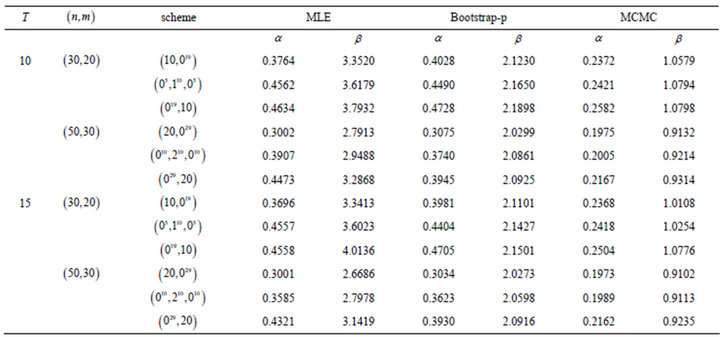
Table 6. The average confidence lengths relative estimate of parameters with
and prior 1.
use the MLEs rather than the Bayes estimators, because the Bayes estimators are computationally more expensive.
NOTES