Effects of Forest Disturbance on Vegetation Structure and Above-Ground Carbon in Three Isolated Forest Patches of Taita Hills ()
Received 6 February 2016; accepted 26 April 2016; published 29 April 2016

1. Introduction
Although forests can mitigate climate change through carbon sequestration and storage (Marland & Schlamadinger, 1997; Chhatre & Agrawal, 2009; Galik & Jackson, 2009) , the contrary is increasingly manifested, particularly in tropical forests as carbon emission through deforestation and forest degradation (Houghton, 2012; Ryan et al., 2012) . For example, tropical deforestation is estimated to have released 1 - 2 billion tonnes of carbon annually during 1990s (Houghton, 2005; Gibbs et al., 2007) . This emission accounted for approximately 15% - 25% of annual global greenhouse gas emissions (Malhi & Grace, 2000; Fearnside & Laurance, 2003, 2004; Houghton, 2005) . In Africa, deforestation accounts for nearly 70% of total emissions (FAO, 2005a, 2005b) . Moreover, CO2 emissions from forest degradation increased significantly, from 0.4 Gt CO2 yr−1 in the 1990s, to 1.1 Gt CO2 yr−1 in 2001-2010 (Federici et al., 2015) and it therefore a critical driver of climate change that requires the deserved attention.
Forests play a critical role in mitigating climate change because of their enormous capacity to sequester and store more carbon when compared to other terrestrial ecosystems (Dale et al., 2001; Ryan et al., 2010; McKinley et al., 2011) . However, when forests are cleared or degraded, the stored carbon is released into the atmosphere as carbon dioxide (CO2), thus negating the aforementioned mitigation.
As the concentrations of atmospheric carbon dioxide continue to increase, scientists and natural resources managers are exploring mitigation options that maximize the amount of carbon stored in terrestrial ecosystems (Malmsheimer et al., 2008) . About 60% of the world’s terrestrial carbon is contained in forest ecosystems, so the response of forests to changes in climate or disturbance regime can have implications for regional and global carbon cycling (Winjum et al., 1992; Dale et al., 2001; Ryan et al., 2010; McKinley et al., 2011) . The amount of carbon stored within a forest does not remain fixed through time. As trees grow and increase in size, the corresponding carbon stocks also increase, and these relationships between forest age and ecosystem carbon pools are well recognized (Otuoma et al., 2016) . Forest carbon stocks typically increase with age until becoming relatively stable after 100 - 150 years, while net ecosystem carbon balance often peaks much earlier and gradually declines to near zero (Pregitzer & Euskirchen, 2004; Bradford & Kastendick, 2010; Williams et al., 2012) . Natural and anthropogenic disturbance events that alter forest stand structure influence site-level carbon stocks and fluxes (Kashian et al., 2006; Gough et al., 2007; Gough et al., 2008; Nave et al., 2010) . Likewise, landscape to regional disturbance regimes or management strategies that alter forest diameter-class distributions over large areas will ultimately drive changes in carbon stocks at landscape to regional levels (Heath & Birdsey, 1993; Pregitzer & Euskirchen, 2004; Mouillot & Field, 2005; Birdsey et al., 2006; Depro et al., 2008; Scheller et al., 2011) .
Already, changes in global climate are occurring (Bernstein et al., 2007) . Due to global changes in climate, natural disturbances are also expected to become more frequent and of higher intensity (Westerling, 2006; Littell et al., 2009; Schelhaas et al., 2010) . Natural disturbances such as forest fires, pests and diseases outbreaks, and extreme weather conditions like El Niño rains typically result in short-term losses in forest carbon stocks, potentially shifting forests from carbon sinks to carbon sources (McKinley et al., 2011; Scheller et al., 2011; Stinson et al., 2011) and potentially influencing climatic conditions via other mechanisms, notably altered albedo (the amount of radiation that is reflected from the Earth’s surface) and energy balance (Randerson et al., 2006; Anderson et al., 2010) . Similarly, the frequency of disturbance across forest areas can also radically alter the potential for carbon storage. In fact at regional scales, increases in disturbance frequency and severity can result in widespread loss of forest carbon stocks (Kurz et al., 2008; Rogers et al., 2011) , while decreases in disturbance frequency are estimated to increase ecosystem carbon stocks by nearly 100% in some regions (Hudiburg et al., 2009) .
Illegal logging for timber and other wood based forest products e.g. fuel wood and poles and encroachment for agriculture are the most common forms of forest disturbance that influence forest carbon stocks by both removing carbon from the ecosystem in form of harvested material and by shifting carbon into detrital pools where it is subsequently returned to the atmosphere through decomposition (McKinley et al., 2011) . Other disturbances include forest fires, grazing and extreme weather conditions such as prolonged droughts and El Niño. All these forms of disturbances occur quite often in Kenya resulting into reduced potential of forests including those found in Taita Hills to store carbon.
Taita Hills form the northeastern part of the Eastern Arc Mountains, a mountain range with an exceptionally high degree of endemism and conservation value (Myers et al., 2000; Burgess et al., 2007; Hall et al., 2009) . These forests of Taita Hills are among the 34 global biodiversity hotspots because of the high number of endemic plant and animal species (Myers et al., 2002; Conservation International, 2005). However, the area of indigenous forest has declined, and becomes fragmented and degraded as a result of deforestation and planting of exotic tree species in degraded sites formerly under indigenous forest (Beentje, 1988; Rogo & Oguge, 2000; Pellikka et al., 2009) . Lately, deforestation of the indigenous forests has been halted and forest conservation and other activities have been introduced in the wake of participatory forest management (Himberg et al., 2009). Although assessment of species biodiversity and similarities between and within the exotic plantations of pine, eucalyptus and cypress, and between the indigenous forest in Ngangao, Chawia and Mbololo forest fragments with the exotic forest in the same forest fragments in the Taita Hills has been done (Rogers, 1996; Omoro et al., 2010) , little is known about the forest structure of Ngangao, Chawia and Mbololo forest fragments and their carbon storage potential.
There are few studies which have been conducted to examine the carbon storage potential of three main forest fragments; Mbololo, Ngangao and Chawia in Taita Hills (Omoro et al., 2013) . The calculation of carbon stock held by individual trees has been done using wood density values for the indigenous species obtained from Reyes et al. (1992) and the global wood density database developed by Zanne et al. (2009) . In cases where the wood density for a species was not listed, an average value of 0.5 was used, as recommended by Chave et al., (2005) for trees from tropical forests. However, none of the tree species from Taita Hills was used in the development of the global wood density database. Inclusion of height and wood density in above-ground biomass (AGB) estimation models has been found to improve on the accuracy in quantifying the carbon stock (Chave et al., 2005, 2014) . However, to-date, studies conducted in fragmented forests of Taita Hills to quantify carbon sequestration potential of the forest fragments have used models with diameter at breast height (DBH) and wood density to estimate the amount of carbon held by these forests (Omoro et al., 2013) leaving out tree height; an important variable in biomass estimation. Improving on the accuracy in quantifying the carbon stock by using models for estimating AGB that integrate diameter at breast height (DBH), wood density and height to calculate the carbon stored by the Mbololo, Ngangao and Chawia forest fragments is therefore a prerequisite in tackling climate change in the context of REDD+ activities (reducing emissions from deforestation and forest degradation and increasing the carbon stock in forests), where governments require more accurate assessment of the forest carbon stocks.
Moreover, no studies have been undertaken to characterize how disturbance can alter forest carbon dynamics over large areas of indigenous forests. Since any attempts to manage and conserve natural forests for enhancing ecosystem carbon stocks must occur in the context of climate change and associated increasing forest disturbances, understanding the simultaneous carbon consequences of both human related and natural disturbances is crucial. To better understand the impact of disturbances on forest carbon stock, it is important to classify sites in each of the forest fragment into heavily, moderately and undisturbed sites and evaluate the impact of different scales of disturbance on the capacity of these forests to sequester carbon. The objectives of this study was to characterize the current forest structure of three forest fragments (Ngangao, Chawia and Mbololo) and ascertain their carbon storage potential in relation to different levels of disturbances.
2. Materials and Methods
2.1. Study Area
The study was undertaken in Taita Hills forests (3˚25'S, 38˚20'E) in Taita Taveta County. Taita Taveta County covers a total surface area of 17,084 Km2 and accounts for 2.94% of Kenya’s total surface area. It borders Tana River, Kitui and Makueni Counties to the North, Kwale and Kilifi Counties to the East, Kajiado County to the Northwest and the Republic of Tanzania to the South and Southwest. The temperature varies between 18.0˚C and 24.6˚C with the Hills experiencing lower temperatures of 18.2˚C compared to the lower zones with an average temperature of 24.6˚C. The average temperature in the Taita Taveta County is 23.0˚C. Taita Hills forests are located within the Inter-tropical Convergence Zone. The long rain season occurs from March to May and a short rain season between November and December, but the mist and cloud precipitation is a year-round phenomenon in the hills. The cloud forest fragments are restricted to areas receiving over 900 mm of annual precipitation, being above 1400 m altitude at the Southeastern slopes and above 1700 m altitude at the Northwestern slopes (Jaetzold & Schmidt, 1983) . The average annual rainfall is 1500 mm. The soils are predominantly cambisols originating from weathered gneiss and are often gravely to sandy-loamy and shallow (Sombroek et al., 1982) . The soils are well-drained and moderately fertile (Sombroek et al., 1982) .
Three forest fragments namely Mbololo, Ngangao and Chawia were covered in this study (Figure 1). These forest fragments were selected because they rank among the most important conservation areas worldwide due to the high concentration of endemic plants and animals (Newmark, 2002; Burgess et al., 2007) . The selected forest fragments have suffered from degradation for over 100 years resulting into isolated patches which are embedded in agricultural rural landscape and are therefore ranked among the most threatened biodiversity hotspots globally (Newmark, 1998; Pellikka et al., 2009) . Mbololo forest (200.0 ha) is the largest continuous forest fragment with low disturbance, Ngangao (120.0 ha) has medium disturbance and Chawia (86.0 ha) has high disturbance. Therefore, the three forest patches provide an exceptional opportunity for assessing effects of forest disturbance on vegetation structure and carbon sequestration potential of Afromontane forests.
2.2. Assessment of Disturbance Levels
A modified method of Sagar et al. (2003) was used to estimate the Disturbance Impact Factor (DIF). The DIF was derived from the relative impact of major disturbance sources or indicators for each of the sites within the forests (Sagar et al., 2003) . Therefore, undisturbed, moderately and heavily disturbed sites within Ngangao and Chawia forests were identified and selected according to modified methods of Oyugi et al. (2007) and Sapkota et al. (2009) . Thus, the number of cut stems, foot paths and trails, timber sawing pits and old logging tracks were counted while crown openness estimated using a densitometer to identify disturbance levels (undisturbed, moderately disturbed and heavily disturbed areas). Footpaths and trails were measured using a linear tape. The relative impact factor of the disturbances (crown openness, cut stems, foot paths and trails, timber sawing pits and old logging) was estimated by assigning either 0 or 1 to the sites within the forests with the lowest number of cases (undisturbed sites) and proportionally higher values to the other forests i.e. moderately and heavily disturbed. By summing the impact factors for each disturbance source, the selected sites within the forests were placed along a disturbance gradient from I (undisturbed) to III (heavily disturbed) (Table 1). The DIF of less than 5 was the reference for undisturbed sites while DIF of greater than 5 but less than 80 was the reference for moderately disturbed sites. The reference DIF for heavily disturbed sites was greater than 80.
2.3. Sampling Design for Data Collection
The forest fragments were naturally occurring subjects having a self-selected level of the independent variable. Mbololo was in totality classified as undisturbed based on the estimated disturbance impact factor (Table 1) while Ngangao and Chawia had sites that were classified as undisturbed, moderately disturbed and heavily disturbed.
Nested plot design with the main plot of 10.0 × 10.0 m was used. The main plot (10.0 × 10.0 m) was used to assess the stand density, carbon stocks, species diversity and frequency. Sub-plots of 2.5 × 2.5 m and 5.0 × 5.0 m were nested within 10.0 × 10.0 m plot for assessment of seedlings and saplings respectively. In Mbololo, all the 32 plots were established in undisturbed sites within forest while in Ngangao, 42, 5 and 9 plots were established in undisturbed, moderately disturbed and heavily disturbed sites respectively for assessment. Additionally, 30, 4 and 5 plots were established in Chawia forest in undisturbed, moderately disturbed and heavily disturbed sites respectively for assessment. Tree species with DBH > 30.0 cm were identified and measured in 10.0 × 10.0 m plots while those with DBH > 2.5 cm < 30.0 cm and DBH < 2.5 cm in the 5.0 × 5.0 cm and 2.5 × 2.5 cm sub-plots respectively. Wood samples were cored from three trees of each of the species represented in the forest having DBH >2.5 cm and taken to the wood anatomy laboratory for analysis of wood density which is also referred to as specific wood gravity.
Stand density was determined by enumerating the number of trees with DBH > 2.5 cm in the 10.0 × 10.0 m plots and multiplying by a factor of 100 to obtain the number of individual trees per hectare. The number of species in the main plots and sub-plots irrespective if their DBH were counted and summed up to determine species richness for each of the forest fragment. The percentage of plots in a given forest fragment in which a species occurred was calculated to determine species frequency. Species diversity was calculated using Shannon-Weiner diversity index (Shannon & Weaver, 1963) using the formula elucidated below:
![]()
Table 1. Estimated disturbance impact factor (DIF) for each disturbance level developed during baseline survey and which was used to classify the sites within the forest fragments as undisturbed, moderately disturbed or heavily disturbed.
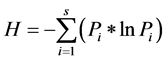
where,
H = Shannon Weiner diversity index
Pi = Fraction of the entire population made up of species i
S = Numbers of species encountered
2.4. Quantification of Above-Ground Carbon Stock
Carbon stocks were calculated according to the biomass models developed by Chave et al. (2005) . The respective models used are:
(1)
(2)
To determine the carbon stock sequestered in relation to forest disturbance, three variables i.e. DBH, tree height and wood density were used using model by Chave et al. (2005) . Consequently, within each 10.0 × 10.0 m plot, one tree with DBH > 10.0 cm was randomly picked for each of the representative species and cored to obtain wood samples and density determined in the laboratory using displacement method. The density (g∙cm−3) determined for each of the tree species together with DBH and height were fitted in allometric models developed by Chave et al. (2005) and used to calculate carbon stocks.
2.5. Determination of Woody Density
The weight of wood cores was measured using a weighing balance to determine the mass in grams and placed into the oven and dried to constant weight at 105˚C. The final constant weight as the oven dry mass was recorded. Using water displacement method, the dried wood core was immersed into a beaker of water loaded on a top-loading electronic weighing balance (Williamson & Wiemann, 2010) . The wood core sample was pressed below the water surface with the aid of a needle and the volume of the wood core on the balance recorded as the volume of the displaced water. Thus, the green volume of the wood core before drying and the dry volume after drying were determined and specific gravity (SG) of wood also referred to as wood density (ρ) calculated using the formula below:
(3)
whereby:
SG = Specific gravity
Oven dry mass = Mass after drying at (101˚C - 105˚C)
ρwater = Density of water which is 1.000 g∙cm−3 at 4.4˚C
2.6. Data Analysis
Data was analyzed using GenStat version 16.0. The data collected was subjected to summary statistics to obtain mean values for stem and seedling densities, species frequency and above ground carbon. Besides, data on stem and seedling densities and above ground carbon was subjected to One-way ANOVA to examine the significance differences in these variables among the three forests. Means that exhibited differences were compared using Tukey’s test with a 5% probability significance threshold.
3. Results
3.1. Stand Density
The stand densities for the forests are presented below (Table 2). As expected the stem density in Chawia and Ngangao forests was high in undisturbed areas compared to moderately and heavily disturbed sites. The stem densities for undisturbed sites were 816.0, 921.9 and 733.3 stems/ha for Chawia, Ngangao and Mbololo forests
![]()
Table 2. Stand densities for Chawia, Ngangao and Mbololo forests at different levels of disturbance.
respectively. For moderately disturbed sites, the density for Chawia forest was 644.4 stems/ha while for Ngangao forest, it was 915.9 stems/ha. Heavily disturbed sites in Chawia forest had a mean density of 444.4 stems/ha while in Ngangao forest, 800.0 stems/ha were recorded. Generally, Ngangao forest had the highest mean stem density of 918.0 stems/ha followed by Chawia forest (896.0 stems/ha) and then Mbololo (733.3 stems/ha). However, there was no significant difference in stem densities for undisturbed, moderately disturbed and heavily disturbed sites in Chawia forest (P = 0.1, LSD = 468.8). The scenario was similar in Ngangao forest, where no significance difference existed among the undisturbed, moderately disturbed and heavily disturbed sites (P = 0.378, LSD = 250.9). The entire Mbololo forest was classified as undisturbed and therefore no comparison was made for the various disturbance levels that occurred as it was the case for Chawia and Ngangao forests.
The seedling density was generally high in Chawia for the different levels of disturbance (Table 3). Disturbed sites had significantly higher number of seedlings (P = 0.039) than undisturbed sites (P = 0.039, LSD = 20528). However, seedling density in moderately and heavily disturbed sites in Chawia did not differ (P > 0.05, LSD = 20528). Undisturbed, moderately and heavily disturbed sites in Ngangao were not significantly different in terms of seedling density (P = 0.363, LSD = 5470). Moderately disturbed sites in Chawia had the highest number of seedlings per hectare (26,400.0) followed by heavily disturbed sites (10,720.0) while undisturbed sites had the least (4255.0). In Ngangao, the situation was different; moderately disturbed sites had the highest seedling density (6933.0 seedlings/ha) followed by undisturbed and heavily disturbed sites with seedling density of 4055.0 and 2667.0 seedlings/ha respectively. In Mbololo where the entire forest was categorized as undisturbed, the seedling density of 2791.0 seedling/ha was lower compared to 4255.0 and 4055.0 seedlings/ha recorded in undisturbed sites in Chawia and Ngangao respectively.
Generally, undisturbed sites had high species diversity than moderately and heavily disturbed sites (Table 4). Considering undisturbed sites, Ngangao had high species diversity (H' = 5.08), followed by Mbololo (H' = 4.99) and then Chawia (H' = 4.46). Moderately disturbed sites in Ngangao forest had high species diversity (H' = 3.02) than Chawia (H' = 1.74). Similarly, heavily disturbed sites in Ngangao had high species diversity (H' = 3.33) than Chawia (H' = 2.35). Unexpectedly, heavily disturbed sites had higher species diversity than moderately disturbed sites in both Ngangao and Chawia forests.
3.2. Species Richness, Frequencies and Levels of Disturbance
Species richness varied among the forests with Mbololo having 39 different species representing 33 genera while Chawia had 28 different species representing 28 genera. Forty two different species were recorded in Ngangao representing 41 genera. The frequency of the species also varied among the forests studied as well as the levels of disturbance (Tables 5-7).
Majority of the species in Chawia were found concentrated in undisturbed forest sites as would be expected in forest sites with minimal disturbance (Table 5). Individual tree species frequencies varied across the disturbance levels with some species such as Tabernaemontana stapfiana, Albizia gummifera, Pleiocarpa pycnantha and Strombosia scheffleri occurring frequently in intact areas than moderately disturbed areas and were absent in heavily disturbed areas (Table 5). This is because the above species are associated with low level of disturbance. In the heavily disturbed sites of Chawia, Rubus volkensii and Betula papyrifera had the highest frequencies because the species are shade intolerant and hence are inclined to heavily disturbed areas with wide open canopies.
In Mbololo, which was not classified as either moderately disturbed or heavily disturbed due to its intactness and therefore the whole forest was categorized as undisturbed, Strombosia scheffleri was the most frequent species followed by Garcinia volkensii, Newtonia buchananii and then Craibia zimmermannii (Table 6). Psychotria taitensis, Rapanea melanophloeos, Syzygium sclerophyllum, Polyscias stuhlmannii, Psychotria crassipetala
![]()
Table 3. Seedlings density for Chawia, Ngangao and Mbololo forests at different levels of disturbance.
![]()
Table 4. Species diversity and the level of disturbance for Chawia, Ngangao and Mbololo forests.
![]()
Table 5. Species frequency for undisturbed, moderately disturbed and heavily disturbed sites in Chawia forest.
![]()
Table 6. Species frequency for undisturbed sites within Mbololo forest.
![]()
Table 7. Species frequency for undisturbed, moderately disturbed and heavily disturbed sites in Ngangao forest.
and Ficus natalensis were the least frequent species in Mbololo. Rubus volkensii, a species associated with heavy disturbance in Chawia was not found to be growing in Mbololo.
Macaranga conglomerata (20.2%) was the most frequent species in the heavily disturbed sites of Ngangao forest followed by Psychotria petitii (15.2%), Cussonia spicata (10.1%), Rapanea melanophloeos (7.1%) and Syzygium guineense (4.0%). Other species frequently occurring in heavily disturbed areas within Ngangao forest included Rytigynia uhligii, Ochna holstii, Albizia gummifera, Ekebergia capensis, Lepidotrichilia volkensii, Maesa lanceolata, Polyscias stuhlmannii, Psychotria crassipetala, Acacia mearnsii, Cupressus lusitanica andPinus patula (Table 7). Macaranga conglomerata, Psychotria petitii, Rapanea melanophloeos and Syzygium guineense were the only occurring all the sites in the forest undergoing three different levels of disturbance. Tabernaemontana stapfiana, Dasylepis integra, Craibia zimmermannii and Cola greenwayi were the main species dominating undisturbed sites of Ngangao forest. In the moderately disturbed sites, Psychotria petitii, Millettia oblata, Oxyanthus pyriformis, Tabernaemontana stapfiana and Newtonia buchananii were found to be the most frequent species. Over and above, some species which were rare in Ngangao could not survive in areas which have experienced moderate and heavy disturbances making them more vulnerable to extinction if the forest continues to be adversely impacted on by anthropogenic activities. Such species include Coffea fadenii, Garcinia volkensii, Leptonychia usambarensis, Podocarpus milanjianus and Xymalos monospora.
3.3. Relationship between Forest Disturbance and Carbon Stock
Carbon stocks for the three forests was calculated using the biomass model comprising of DBH, H and ρ because the model has been proved to be more accurate than models that utilizes DBH and ρ to quantify carbon stock in tropical forests (Chave et al., 2005, 2014) . Even though there was no significant difference among undisturbed, moderately and heavily disturbed sites in Chawia (P = 0.616, LSD = 868.7) and Ngangao (P = 0.652, LSD = 599.4), it is evident that forest disturbance reduced the capacity of the forests to sequester carbon (Table 8). Moderate disturbance reduced the capacity of Chawia forest to sequester carbon by 38.8% or 169.0 Mg∙ha−1 while heavy disturbance reduced carbon sequestration potential of Chawia by 70.7% or 307.8 Mg∙ha−1. Moderately disturbed sites in Ngangao sequestered 376.5 Mg∙ha−1 of carbon compared to 414.5 Mg∙ha−1 in undisturbed sites. Thus, the quantity of carbon sequestered by moderately disturbed sites in Ngangao was 9.2% or 38.0 Mg∙ha−1 less what the undisturbed sites were seizing. Moreover, heavily disturbed sites in Ngangao sequestered 65.7% or 272.3 Mg∙ha−1 less carbon compared to undisturbed sites. It is eminent from the present study that heavily disturbed sites in Chawia (70.7%) and Ngangao (65.7%) lost almost the same capability to sequester above ground carbon. By and large, Chawia had the highest quantity of carbon stock for the undisturbed sites (435.5 Mg∙ha−1) followed by Ngangao forest (414.5 Mg∙ha−1) while Mbololo forest was found to sequester the least amount of carbon per unit area (261.2 Mg∙ha−1).
3.4. Accuracy of Biomass Models in Estimation of Carbon Stock
Models for estimating biomass which use diameter at breast height (DBH), height (H) and wood density (ρ) have been found to provide more accurate above-ground carbon estimates than those with DBH and ρ and DBH alone (Chave et al., 2005, 2014) . Our results indicate that the use of models with DBH and ρ to estimate carbon gave higher quantities of carbon sequestered in natural forest ecosystems than models with DBH, height and wood density (Figure 2). The estimated quantity of carbon sequestered in undisturbed sites in Chawia was 561.2 Mg∙ha−1 when the DBH and ρ model was used whereas the model with DBH, H and ρ gave carbon stocks estimates of 435.5 Mg∙ha−1. Similar trend was observed for moderately disturbed sites in Chawia where estimates of 341.5 Mg∙ha−1 and 266.5 Mg∙ha−1 were recorded for DBH and ρ and DBH, H and ρ models respectively. However, in heavily disturbed sites, both models gave carbon estimates that were more or less similar, 127.7 and 126.5 Mg∙ha−1 for DBH and ρ and DBH, H and ρ models respectively.
Similar to the findings in Chawia, the trend in the quantity of carbon estimated in Ngangao was higher for biomass model with DBH and ρ than models that had DBH, H and ρ as variables in the equation (Figure 3). In undisturbed sites, DBH and ρ based model estimated the quantity of carbon sequestered per unit hectare to be 571.7 Mg∙ha−1 while the model with DBH, H and ρ gave carbon estimates of 414.5 Mg∙ha−1. Carbon quantities of 488.2 Mg∙ha−1 (DBH and ρ model) and 376.5 Mg∙ha−1 (DBH, H and ρ model) were calculated in moderately disturbed sites of Ngangao. Heavily disturbed sites in Ngangao unlike Chawia showed strident differences in the amount of carbon sequestered using the different models. While the amount of above ground carbon sequestered
![]()
Table 8. Above ground carbon at different levels of disturbance.
![]()
Figure 2. The quantity of carbon estimated in Chawia forest using two different biomass models at different levels of disturbance; error bars represents standard error of mean.
![]()
Figure 3. The quantity of carbon estimated in Ngangao forest using two different biomass models at different levels of disturbance; error bars represents standard error of mean.
by Ngangao in heavily disturbed sites was estimated to be 244.7 Mg∙ha−1 when DBH and ρ based model was used, the estimated above ground carbon decreased to 142.2 Mg∙ha−1 when DBH, H and ρ model was used to quantify carbon.
Mbololo did not have sites that could be classified as moderately and heavily disturbed since the entire forest was categorized as an intact forest. Many authors (Beentje, 1988; Pellika et al., 2009; Omoro et al., 2010) have all reported that Mbololo remains undisturbed due to its strategic location. Unlike Chawia and Ngangao which are located in the middle of agricultural landscape, Mbololo is not surrounded by agricultural landscapes and also, is not easily accessible due to poor road network. Besides, the communities living around Mbololo forest have strong cultural beliefs that discourage locals from engaging in forest destructive activities. The forest is also far away from urban centers where the demand for products such as charcoal, poles and timber is high. All these factors have worked concurrently to keep Mbololo forest intact (C. Wekesa, Kenya Forestry Research Institute, Personal Communication, 2016). Therefore, testing of the accuracy of the models in estimating carbon sequestered by the forest was only based on the undisturbed sites. The results of carbon estimates for Mbololo using the different models followed the same trend as Chawia and Ngangao forests for the undisturbed sites (Figure 4). In Mbololo, the model with DBH and ρ gave carbon quantities of 435.3 Mg∙ha−1. However, when the model consists of DBH, H and ρ was used to guesstimate carbon sequestered by Mbololo forest, the quantity of carbon reported was 261.2 Mg∙ha−1. It was found that the model with DBH and ρ substantially gave higher quantities of carbon sequestered by 174.1 Mg∙ha−1 in Mbololo.
The application of DBH and ρ based model to quantify above ground carbon was found to give higher values of carbon stocks by between 0.9% and 44.4% compared to DBH, H and ρ model depending on the site (forest fragment), level of disturbance and stand density (Table 9). In Chawia where the stand density was 816.0 and 644.4 stems/ha for undisturbed and moderately disturbed sites respectively, DBH and ρ based model yielded higher quantities of carbon by 22.0% and 22.4% for undisturbed and moderately disturbed sites respectively than carbon stocks estimated using DBH, H and ρ model. The carbon sequestered in heavily disturbed sites of Chawia was 0.9% higher for DBH and ρ based model compared to the quantities estimated by DBH, H and ρ model and this estimation was smaller compared to undisturbed and moderately disturbed sites. The trend for Ngangao for undisturbed and moderately disturbed sites was similar to what was observed in Chawia whereby carbon stocks were 22.9% and 27.5% higher for undisturbed and moderately disturbed sites respectively when
![]()
Figure 4. The quantity of carbon estimated in Mbololo forest using two different biomass models for undisturbed sites; error bars represents standard error of mean.
![]()
Table 9. Over-estimation of carbon stock resulting from the use of DBH and wood density model as compared to DBH, height and wood density model for Chawia, Ngangao and Mbololo forests at different levels of disturbance.
DBH and ρ based model was used. However, for heavily disturbed sites, carbon reported when DBH and ρ model was used was very high in Ngangao forest (41.9%) compared to Chawia forest (0.9%). Comparing the results for undisturbed sites across the three forests, the highest quantity of carbon sequestered when DBH and ρ model was used in calculating above ground carbon was found to be in Mbololo (40.0%).
4. Discussion
Our results suggest that different levels of disturbance were responsible for the substantially lower stem density in the disturbed sites within the forests which have been subjected to moderate and heavy disturbance. Although the stem density of trees with diameter at breast height (DBH) greater than 2.5 cm was significantly higher in undisturbed sites than moderately and heavily disturbed sites, the seedling density was higher in the moderately disturbed sites than heavily disturbed sites in Ngangao and Chawia forests. Stand density for trees (DBH > 2.5 cm) for undisturbed, moderately disturbed and heavily disturbed sites in the three forests did not deviate from what was reported in the previous studies (Rogers et al., 2008; Omoro et al., 2010) despite slight differences in the minimum threshold value of DBH used in the studies. Regarding seedling density, Chawia exhibited the highest seedling density for both moderately and heavily disturbed sites than Ngangao. Forest disturbances have been observed to stimulate regeneration of diverse species through intermediate succession stages (Hobbs & Huenneke, 1992; Chazdon, 1998) and hence in Chawia, such disturbances could have favoured growth of secondary indigenous species which are associated with low levels of disturbance such as Tabernaemontana stapfiana, Xymalos monospora, Syzygium guineense and Albizia gummifera (Omoro et al., 2010) . Sapkota et al. (2009) previously reported that the density of advanced regeneration was higher in the moderately disturbed forests than in either the least or heavily disturbed forests as confirmed by the present study. Moreover, the possible presence of soil seed bank associated with initial clearance of the forests (Wassie & Tekatay, 2005) , may explain the highest level of regeneration of the indigenous species in Chawia forest. In contrast to Chawia, the gradient or slope for Ngangao is more steep leading to seeds of some species being washed away during heavy downpour and thus encumbering germination. Hindered germination of seeds deposited on the forest floor in Ngangao was observed to be contributing to low regeneration rate especially for species such as Psychotria petitii and this is in agreement with findings by Eilu and Obua (2005) and could also explain why the seedling density was higher in Chawia than Ngangao.
Surprisingly, Chawia which according to Pellikka et al. (2009) is the most disturbed among the three forests, had higher overall stem density for trees with DBH > 2.5 cm followed by Ngangao (moderately disturbed) and Mbololo (least disturbed). This is could be attributed to vigorous regeneration and ensuing recruitment of regenerates (saplings) into trees with DBH > 2.5 cm leading to increased stand density. The presence of high densities of secondary species associated with disturbance like Tabernaemontana stapfiana, Xymalos monospora, Syzygium guineense and Albizia gummifera could also have helped the situation given that these species are fast growing and hence recruited into the next stage within a short time compared to other indigenous species like Strombosia scheffleri, Craibia zimmermannii and Newtonia buchananii which are not typically associated with disturbance (Aerts et al., 2011) . Consequently, the current results indicate that the conditions for Chawia have improved for the last 5 years when benchmarked to the earlier reports (Pellikka et al., 2009; Omoro et al., 2010) to almost match Mbololo which is undisturbed.
There was striking differences in species richness among the forests which could be attributed to the recurring anthropogenic activities such as collection of firewood, illegal logging as well as trampling which substantially altered species habitats as earlier reported by Pandey & Shukla (1999). Therefore, the species richness of forest sites subjected to disturbance depends on the differential responses of individual species to such disturbances; some species may tolerate the disturbances, while others may become locally extinct (Sagar et al., 2003) . Species such as Tabernaemontana stapfiana, Albizia gummifera, Pleiocarpa pycnantha and Strombosia scheffleri had limited ability to cope with heavy disturbance leading to their disappearance in the sites within the forests that were heavily disturbed. Consequently, these species could be on the road to extinction if the current rate of forest degradation continues unabated and this could lead to austere consequences including loss of biodiversity and reduced capacity of the forests to provide essential ecosystem services like carbon sequestration. In contrast, Rubus volkensii and Betula papyrifera were able to cope with heavy disturbance and could be excellent candidate species for selection in rehabilitation and restoration of heavily disturbed sites characterized by open canopies. Within each forest fragment, the species richness varied with disturbance levels (undisturbed, moderately and heavily disturbed sites). As expected, the species richness was higher in undisturbed sites than moderately and heavily disturbed sites. However, heavily disturbed sites in Ngangao and Chawia forests had higher species richness than moderately disturbed sites. Earlier studies have shown that heavy disturbances in forests can kill mature trees, but also create conditions necessary for the establishment of new tree cohorts and create microhabitats for new plant and animal species, thereby increasing the species diversity compared to less or moderately disturbed stands (Fischer et al., 2013) . Therefore, heavy forest disturbance generated favourable micro-envi- ronment that facilitated the emergence of new tree species that enhanced the species richness in heavily disturbed sites.
Over and above, species richness varied among the forests. Ngangao had the highest species richness followed by Mbololo. Species richness was lowest in Chawia. The low species richness observed in Chawia could be explained by the high level of disturbance the forest has undergone for several decades mainly due to anthropogenic activities as reported by Pellikka et al. (2009) and Omoro et al. (2010) . Despite being classified as moderately disturbed (Pellikka et al., 2009) , Ngangao had high species richness than Mbololo which is least disturbed. Theabsence of seed banks in Mbololo (Omoro et al., 2010) could have stalled regeneration which may have contributed significantly to the reduced species richness compared to Ngangao. Besides, Mbololo showed little regeneration resulting to low stand density compared to Ngangao. Species richness and stand density in the intact mature forest has been found to be significantly less than that in the disturbed forest (Huang et al., 2003) and hence, the low stand density in Mbololo was responsible for the reduced species richness.
As it would be expected, undisturbed sites had high species diversity than moderately and heavily disturbed sites. The species diversity for undisturbed sites was higher in Ngangao followed by Mbololo while Chawia had the least species diversity. In the case of moderately disturbed sites, high species diversity was observed in Ngangao compared to Chawia forest. Similarly, for the case of heavily disturbed sites, Ngangao had high species diversity than Chawia forest. Omoro et al. (2010) reported that species diversity was higher in Mbololo followed by Ngangao and then lastly Chawia and this has been confirmed by these findings particularly for moderately and heavily disturbed sites. However, the Shannon Weaver Indices reported in the current study were higher than what was reported by Omoro et al. (2010) which is attributed to different ecological assessment methods used. While Omoro et al. (2010) enumerated trees with minimum DBH of 5.0cm; this study used a threshold value of 2.5cm. Unexpectedly, heavily disturbed sites had higher species diversity than moderately disturbed sites in both Ngangao and Chawia forests. This could be credited to substantial regeneration of species that are able to thrive in forest gaps due to the opening of the forest canopy. Ecologically, forest gaps have been found to contribute to the maintenance of high species diversity in tropical forests and are therefore significant in forest ecosystem management (Egbe et al., 2012) .
Generally, forest disturbance altered the forest stand structure thereby influencing site-level carbon stocks and this is consistent with results reported in numerous studies (Gough et al., 2007; Gough et al., 2008; Nave et al., 2010) . Moderate disturbance reduced the capacity of the forest fragments to sequester carbon by between 9.2% and 38.8% while heavy disturbance reduced carbon sequestration potential of the forest fragments by between 65.7% and 70.7% depending on the forest fragment under consideration. The impact of heavy disturbance on capacity of the forests to sequester above ground carbon was almost the same given the insignificant difference of 5.0% observed between heavily disturbed sites in Ngangao and Chawia. However, the effect of moderate disturbance on the capacity of the forest fragments to sink carbon varied significantly between different forest fragments as revealed in the gigantic range in percentage loss in carbon sequestered in Ngangao and Chawia. According to Bradford et al. (2013) natural disturbance and logging exert stronger influence on forest carbon stocks. Moreover, it has been found that under low natural mortality, forest-wide total ecosystem carbon stocks increased between 0% and 40% when planned harvests were implemented; however, carbon stocks decreased with greater harvest levels (>40%) and elevated disturbance rates (McKinley et al., 2011; Bradford et al., 2013) .
The quantity of carbon sequestered varied among the forest fragments for the undisturbed, moderately and heavily disturbed sites. In the case of undisturbed sites, Chawia had higher quantities of carbon stock followed by Ngangao then Mbololo. Moderately and heavily disturbed sites in Ngangao had more carbon than Chawia. These differences in carbon stocks were attributed to differences in the species diversity, richness and frequency. Tree species in tropics exhibit different growth rate and patterns and as a result they vary considerably in terms of size (DBH and height) and specific wood gravity or wood density. These variables i.e. DBH, height and wood density determines the amount of carbon a tree can store. Due to high diversity of woody species in Ngangao, Mbololo and Chawia, the DBH, height and wood density varied greatly resulting into differences in carbon stocks sequestered by the forest fragments. The variation in species frequency would also be a source of variability in carbon estimates.
Omoro et al. (2013) reported a mean carbon stock of 360 Mg∙ha−1 for indigenous forests of Taita Hills (Ngangao, Chawia, Mbololo and Irizi). Similarly, studies in Kakamega forest by Glenday (2006) reported a mean carbon stock of 360 Mg∙ha−1 for indigenous forest. Our values for carbon stock per unit area differed with findings of Omoro et al. (2013) and Glenday (2006) . While Omoro et al. (2013) and Glenday (2006) did not categorize the forests into sites exhibiting different levels of disturbance, this study classified the areas within the forest fragments into undisturbed, moderately and heavily disturbed sites and this explains the difference in the results. However, the quantity of carbon sequestered of 376.5 Mg∙ha−1 by moderately disturbed sites in Ngangao compares well with results reported by Omoro et al. (2013) and Glenday (2006) .
Compared to the model with DBH, H and ρ, the use of DBH and ρ based model to quantify above ground carbon was found to yield higher quantities of carbon stock by between 0.9% and 44.4% depending on the site (forest fragment), level of disturbance and stand density. This is despite Chave et al., 2005 ranking the two models as being the overall best models, depending on whether total tree height is available or not. Previous studies (Brown & Lugo, 1982; Malhi et al., 2004) have indicated that uncertainties in the estimation of biomass carbon stocks of tropical forests resulting from the use of models without height and wood density prohibit accurate assessment of carbon quantities sequestered which is consistent with the findings of the present study. Consequently, models for estimating biomass consisting of three variables i.e. diameter at breast height (DBH), height (H) and wood density (ρ) provide more accurate above-ground carbon estimates than those with DBH alone (Chave et al., 2014) . Therefore, successful implementation of REDD+ and similar mechanisms could certainly be achieved if models with DBH, H and ρ are used in estimating biomass because they have been proved to provide reliable estimation of biomass carbon stocks in tropical forests (Petrokofsky et al., 2012) . However, the huge differences of quantities of carbon estimated by models with DBH, H and ρ and those with DBH and ρ is a strong pointer that locally developed allometric equations for specific natural forest ecosystems are likely to give more accurate biomass estimates than Tier 2 and Tier 3 biomass models. Efforts should therefore be directed towards developing local biomass equations for each of the unique forest ecosystems to improve on the accuracy of carbon stocks estimation.
5. Conclusion
Different scales of disturbance had altered the forest structure, reduced species diversity and decreased the capacity of the forest fragments to sequester carbon. Moderate and heavy forest disturbances resulted in low stand density compared to undisturbed sites within the same forest fragment. Further, moderately and heavily disturbed sites had low species diversity 40% - 50% less compared to undisturbed sites indicating that anthropogenic activities’ driven disturbance greatly threatens biodiversity conservation. Reassuringly, moderately and heavily disturbed sites had high rate of species regeneration which if well protected could lead to recovery of these sites and restore the ecological integrity of the forest fragments to effectively provide ecosystem services including carbon sequestration.
Key indigenous species such as Tabernaemontana stapfiana, Craibia zimmermannii, Dasylepis integra, Cola greenwayi, Albizia gummifera, Pleocapa pycanatha and Strombosia scheffleri were found in undisturbed sites but were missing or rare in moderately and heavily disturbed sites. Consequently, disturbance is likely to condemn these species to extinction and hence synchronized effort is required to halt disturbance if these species and the associated biodiversity are to be conserved and hoarded from extinction.
The capacity of the forest fragments to sequester atmospheric carbon was greatly reduced because of forest disturbance. Between 9.2% and 70.7% of carbon stock which could otherwise have been seized by these forest fragments was lost due to disturbance. The reduced capacity of these forests to sequester carbon is a bottleneck in tackling climate change through REDD+, a strategy by UNFCCC aimed at reversing degradation and increasing forest cover. Participatory rehabilitation of the disturbed sites with indigenous endemic tree species could be the starting point to enhance the forests’ capacity in mitigating against climate change through carbon sequestration.
In comparison with DBH, H and ρ, the use of DBH and ρ models to estimate above ground carbon overestimated the carbon stocks by between 0.9% and 44.4% depending on the site (forest fragment), level of disturbance and stand stem density. Therefore, model with diameter at breast height (DBH), height (H) and wood density (ρ) could be more accurate in estimating carbon stock in tropical forests and hence reliable. Since governments require accurate data on forest carbon stocks and carbon stock changes, models with diameter at breast height (DBH), height (H) and wood density (ρ) are recommended for estimating carbon stock in natural forests. Nevertheless, to minimize bias and improve the accuracy of biomass assessment, development of locally derived ecosystem based diameter-height-wood density allometric equations is advised.
Acknowledgements
The authors would like to thank Kenya Forestry Research Institute (KEFRI) for funding this study through Forest Biodiversity and Environment Management thematic area. Special thanks to Kenya Forest Service, Wundanyi station for their support during setting up of the studies and data collection. We also thank the field crew: Joseph Muthini, Jonam Mwandoe, Gabriel Mwarabu and John Mbori for their effort during data collection. Thanks to Regional Director and Deputy Regional Director, KEFRI Coast Eco-Region Research Programme for their technical guidance and facilitating this work.
NOTES
![]()
*Corresponding author.