Classification of Emphysema Subtypes: Comparative Assessment of Local Binary Patterns and Related Texture Features ()
1. Introduction
Chronic obstructive pulmonary disease (COPD) is characterized by persistent airflow limitation that is usually progressive and associated with an enhanced chronic inflammatory response in airways and lungs, which is caused by cigarette smoke and other noxious particles [1] . This chronic inflammatory response may induce parenchymal tissue destruction (resulting in emphysema), and disrupt normal repair and defense mechanisms (resulting in small airway fibrosis). According to the projection from 2002, COPD is predicted to become the fourth leading cause of death in the world by 2030 [2] .
Pulmonary function test (PFT) is a non-invasive examination, which provides valuable information for evaluation of COPD. For example, based on forced expiratory volume in one second (FEV1), severity of airflow limitation is divided into four grades in COPD [1] . However, FEV1 is an unreliable marker of severity of breathlessness, exercise limitation, and health status impairment at an individual patient level [1] . Therefore, reliable method for evaluating COPD should be developed.
Pulmonary emphysema is visually classified into three subtypes: centrilobular emphysema, panlobular emphysema, and paraseptal emphysema [3] . Centrilobular emphysema is the most common subtype of emphysema, and is strongly associated with cigarette smoking, whereas panlobular emphysema is often seen with alpha1-anti- trypsin deficiency [4] . Paraseptal emphysema appears to be important in the development of spontaneous pneumothorax, but is not less associated with airflow obstruction [4] . Considering these differences of emphysema subtypes, the subtype classification would make it possible to accurately evaluate airflow limitation caused by emphysema.
Computed tomography (CT) has been used in order to assess extent of emphysema. Goddard et al. [5] proposed scoring system for visual assessment of pulmonary emphysema, and the scoring system had been utilized in previous study [6] . However, visual assessment of emphysema is subjective and time-consuming, and suffers from inter-observer variability [7] . For example, kappa values for detection of centrilobular emphysema (CLE), panlobular emphysema, and paraseptal emphysema (PSE) ranged from 0.29 to 0.59 [7] , and the inter-observer variability in emphysema subtypes was not acceptable. Under these circumstances, importance of automated CT quantification of emphysema has been recognized, which provides objective and reproducible evaluation of COPD. Recently, many researchers have begun to show the usefulness of texture analysis to classify small-sized regions in CT images [8] - [16] . Sørensen et al. [8] showed that quantitative assessment of emphysema subtypes could be performed using texture features such as local binary patterns (LBP) [17] . Using the classification of emphysema subtypes, relationship between emphysema subtypes and airflow limitation could be investigated more precisely in COPD patients.
Although CT quantification is a promising tool for emphysema evaluation, radiation exposure is one of the major limitations in CT. To decrease potential cancer risk of radiation exposure, low-dose CT has been performed increasingly. In addition, reflecting the current worldwide interest in CT-based lung cancer screening [18] , much attention has been paid to emphysema quantification on low-dose CT to stratify lung cancer risk [19] . In line with this trend, there was a recent study showing that degree of emphysema assessed on low-dose CT was a predictor of death from COPD and lung cancer [20] .
To the best of our knowledge, there has been no attempt to classify emphysema subtypes on low-dose CT images using LBP and related texture features. Thus, the purpose of this study was to comparatively assess the usefulness of LBP and related texture features, namely completed local binary patterns (CLBP) [21] and local ternary patterns (LTP) [22] , for the classification of emphysema subtypes on low-dose CT images. LBP was introduced and promoted by Ojala et al. [17] , and CLBP and LTP were developed as extensions of LBP. LBP is a local texture descriptor, whose advantages are computational simplicity, robustness against gray-scale variations, and that against rotation variations. In CLBP, local texture is represented by three components (sign component, magnitude component, and center gray level), and is more discriminant than LBP [21] . While LTP is also a discriminant local texture descriptor, LTP is less sensitive to image noise than LBP [22] .
2. Materials and Methods
The review board of our institution approved this retrospective study. Written informed consent was obtained from all patients for the use of their data.
2.1. Patients
Between October 2009 and August 2011, fifty patients (34 men and 16 women; mean age ± standard deviation, 67.5 ± 10.1 years) who underwent low-dose CT scans were included in this study. They underwent the CT scans for screening or stating of lung cancer. The smoking history and predicted values of FEV1 of these patients were 38.2 ± 34.3 pack-years and 84.5% ± 19.5%, respectively. The patients consisted of 17 never smokers, 13 smokers without COPD, and 20 smokers diagnosed with COPD based on the Global Initiative for Chronic Obstructive Lung Disease criteria [1] . In the COPD smokers, predicted values of FEV1 was 69.5% ± 17.9%.
2.2. CT Scan Protocol
Each patient was scanned with a 320-detector-row scanner (Aquilion ONE; Toshiba Medical Systems, Otawara, Japan), and non-contrast helical CT scans were acquired from the lung apices through the lung bases. The scan parameters of low-dose CT were as follows: tube current, 120 mA; tube potential, 120 kV; gantry rotation time, 0.5 second. Raw CT data were reconstructed into contiguous 1-mm-thick images with a standard lung kernel (FC 51) by using filtered back projection.
2.3. Selection of ROI
Two board-certified radiologists, who had 11 and 6 years of experience as chest radiologists, selected 3681 nonoverlapping regions of interest (ROIs) from low-dose CT images. Then, by consensus reading of the two radiologists, they annotated these ROIs as belonging to one of the following three classes: normal tissue (NT), CLE, and PSE. Here, NT ROIs were selected from never smokers, and CLE and PSE ROIs were selected from smokers without COPD or smokers with COPD. Because there were few regions of panlobular emphysema, panlobular emphysema was excluded from analysis in this study. Thus, 1352 NT ROIs, 1269 CLE ROIs, and 1060 PSE ROIs were obtained. Representative images of NT, CLE, PSE ROIs are shown in Figure 1.
2.4. Image Feature Vector of ROI
In this study, the annotated ROIs were represented as image feature vector, which consisted of CT density histogram and texture histogram. The schematic illustration of calculation of image feature vector is shown in Figure 2.
To obtain CT density histogram of the ROI, CT densities of all the pixels within the ROI were collected. Then, using histogram bins, CT density histogram of the ROI was calculated. The histogram bins were obtained by dividing the range (−1000 to 1000 HU) by number of histogram bins (G).
(a) (b) (c)
Figure 1. Representative images of ROI annotated by the radiologists. (a) Normal tissue; (b) Centrilobular emphysema; (c) Paraseptal emphysema.
![]()
Figure 2. Schematic illustration of calculation of image feature vector. Abbreviations: local binary patterns (LBP), completed local binary patterns (CLBP), local ternary patterns (LTP).
After obtaining the histogram of CT densities, the 3 types of texture histograms (LBP, LTP, and CLBP) were calculated as previously reported [17] [21] [22] . LBP takes a local neighborhood around each pixel, thresholds the neighbor pixels at the value of the center pixel, and uses the resulting binary-valued image patch as a local image descriptor. Formally, the LBP operator is represented as follows:

where x is the center pixel where LBP is calculated; P is number of samples; R is radius; n(x, R, i) is the ith neighbor pixel around the center pixel x and the distance between the center pixel x and the neighbor pixel is R; I(u) is CT density of pixel u; s(v) is an indicator function, where s(v) is1 if v ≥ 0 and 0 otherwise. In our study, rotation invariant LBP were used [17] . LBP operator was applied in each pixel of the ROI, and LBP histogram was obtained by collecting the results of LBP operator within the ROI.
In LTP, the indicator function of LBP is replaced by a 3-valued function:
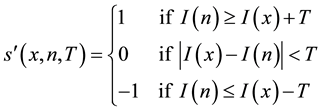
where T is threshold. In LTP,
is applied to the center pixel x and neighbor pixel n, where n is determined by R and P as LBP. Then, the resultant 3-valued ternary patterns is divided into its positive and negative parts. Finally, these two parts are treated as two separate channels of LBP descriptors for which separate two histograms are computed, combining these at the end of the computation of LTP texture histogram [22] .
In CLBP, the local neighborhood is represented as the 3 components: CLBP_C, CLBP_S, and CLBP_M [21] . CT density of center pixel of local neighborhood represents the image gray level, and CT density distribution of center pixels is converted into CLBP_C. On the other hand, image local differences of local neighborhood are also utilized in CLBP, and the local differences are divided into two complementary components: sign and magnitude components. Using the sign components, the CLBP_S can be calculated, which is identical to the original LBP operator. Using the magnitudes components and global threshold, CLBP_M is calculated. By combining the CLBP_C, CLBP_S, and CLBP_M, CLBP texture histogram can be obtained.
After obtaining the CT density histogram and texture histogram, the CT density histogram and 3 types of texture histograms were concatenated. In the current study, the CT density histograms and texture histogram were represented as one-dimensional vector. Here, CT density histogram (D) and texture histogram (T) are denoted as follows:
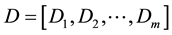
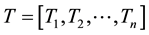
where m and n are lengths of CT density histogram and texture histogram, respectively. Then, the resultant combined histogram (H) is represented as follows:

where length of the resultant histogram (H) is m + n. In other words, concatenation of two types of histograms is performed to obtain combined histogram. Using the CT density histogram, LBP, CLBP, and LTP, the 3 types of the combined histograms were obtained (hereafter referred to as combined LBP, combined CLBP, and combined LTP). These 3 types of the combined histograms were used as image feature vectors to classify the ROIs.
2.5. Parameters and Classification
The ROIs were classified based on the image feature vector represented as combined LBP, combined CLBP, or combined LTP. For calculation of image feature vector of ROI, the following parameters and their combinations were used: number of histogram bins (for CT density histogram) G = {20, 25, 30, 35, 40}; R = {1, 3, 5, 7, 9} pixels; P = {8}; T = {10, 20, 40, 80} Hounsfield units (for combined LTP only); and ROI size = {7 × 7, 11 × 11, 13 × 13, 19 × 19} pixels. While P = 16 was frequently used for LBP in the past studies, the computational cost when using P = 16 in LBP, CLBP, and LTP was unacceptable in the current study. Therefore, only P = 8 was used. Linear support vector machine (SVM) was used for ROI classification, whose implementation was freely available [23] . Hyperparameters of linear SVM were {2−10, 2−9, 2−8, …, 27, 28, 29}.
2.6. Statistical Analysis
The accuracy of classification was determined by patient-based 10-fold cross validation for every combination of image feature type, G, R, P, ROI size, and hyperparameter of linear SVM. In the patient-based cross validation, to avoid the situation that ROIs obtained from one patient were divided into training data and test data, all the ROIs from one patient belonged to either training data or test data. In combined LTP, effect of T was also investigated. Accuracy is defined as the following equation:
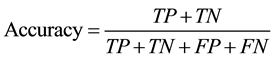
where TP, TN, FP, and FN are numbers of true positives, true negatives, false positives, and false negatives, respectively. Based on the results of the cross validation, the optimal value of classification accuracies were selected for each type of image feature vector. In addition, using the optimal combination of the parameters, the contingency table of ROI classification was calculated.
3. Results
For combined LBP, combined CLBP, and combined LTP, the accuracies of ROI classification are shown in Figures 3-5. Tables 1-3 show the contingency table of combined LBP, combined CLBP, and combined LTP, when using the optimal combinations of the parameters.
![]()
Figure 3. Accuracies of ROI classification obtained with combined LBP. Abbreviation: local binary patterns (LBP).
![]()
Table 1. Contingency table of the ROI classification using combined LBP.
Note: The accuracy of the classification was 81.36%. The parameters were: ROI size = 7 × 7, G = 25, R = 9, P = 8. Abbreviations: local binary patterns (LBP), normal tissue (NT), centrilobular emphysema (CLE), araseptal emphysema (PSE).
![]()
Figure 4. Accuracies of ROI classification obtained with combined CLBP. Abbreviation: completed local binary patterns (CLBP).
![]()
Figure 5. Accuracies of ROI classification obtained with combined LTP Note: in combined LTP, the best accuracy was selected from the results corresponding to T = {10, 20, 40, 80} Hounsfield units. Abbreviation: local ternary patterns (LTP).
![]()
Table 2. Contingency table of the ROI classification using combined CLBP.
Note: The accuracy of the classification was 82.99%. The parameters were: ROI size = 19 × 19, G = 25, R = 9, P = 8. Abbreviations: completed local binary patterns (CLBP), normal tissue (NT), centrilobular emphysema (CLE), araseptal emphysema (PSE).
![]()
Table 3. Contingency table of the ROI classification using combined LTP.
Note: The accuracy of the classification was 83.29%. The parameters were: ROI size = 19 × 19, G = 30, R = 9, P = 8, T = 80. Abbreviations: local ternary patterns (LTP), normal tissue (NT), centrilobular emphysema (CLE), araseptal emphysema (PSE).
The best accuracy of combined LBP, combined CLBP, and combined LTP were 81.36%, 82.99%, and 83.29%, respectively. Compared to the classification accuracies obtained with combined LBP, those with combined LTP or combined CLBP were consistently improved. % also show that the accuracies of classification were improved by using larger R. The optimal combinations of the parameters were: for LBP, ROI size = 7 × 7, G = 25, R = 9, P = 8; for CLBP, ROI size = 19 × 19, G = 25, R = 9, P = 8; and for LTP, ROI size = 15 × 15, G = 30, R = 9, P = 8, T = 80.
4. Discussion
To our knowledge, this was the first study to classify the emphysema subtypes on low-dose CT using LBP, CLBP, and LTP. Compared to combined LBP, the subtype classification was improved with combined CLBP and combined LTP. According to these results, the classification of emphysema subtypes was performed successfully on low-dose CT, and our methodology to classify emphysema subtypes was validated.
Although percentage of low-attenuation volume is commonly used as emphysema quantification [6] [24] , it ignores the emphysema subtypes. To precisely investigate the relationship between emphysema subtypes and airflow limitation, extent of emphysema subtypes should be evaluated. Although it is possible to assess emphysema subtypes visually, the visual assessment was time-consuming and suffered from inter-observer variability [7] . To overcome these problems, automated CT quantification of emphysema subtypes should be pursued, which would provide additional benefits with radiologists. In this study, it was shown that the ROI-based classification of emphysema subtypes was successfully performed. Using the same methodology, emphysema subtypes of entire lungs can be analyzed in COPD patients. We expect that our methodology can provide useful information about emphysema subtypes on low-dose CT.
Noise is inevitably present on CT images, especially on low-dose CT images. In general, as lower radiation dose is used, the noise on CT images increases. Due to relatively high level of image noise, it is difficult to capture texture information accurately on low-dose CT images. Because of the threshold (T) in LTP, texture analysis using LTP is expected to be more robust to noise than that using LBP. Intuitively, T must be chosen according to noise level and texture characteristics. If T is too large, texture information cannot be encoded into LTP histogram efficiently. On the other hand, if T is too small, the image noise affect local texture substantially. Therefore, optimal threshold for LTP must be chosen to balance the noise level and the characteristics of texture. In the present study, four different thresholds were used for calculating LTP, and combined LTP slightly outperformed combined LBP. These results indicate that LTP was robust to the image noise caused by dose reduction when the optimal value of T was selected.
In CLBP, local texture is represented by three components, and one of them (sign component) is identical to the original LBP. Compared with LBP, CLBP can capture local texture information more robustly by utilizing the other two components. We speculate that, because of this property of CLBP, combined CLBP was superior to combined LBP in the current study.
According to Figures 3-5, the classification accuracies were improved by using larger values for the radii (R) of LBP, CLBP, and LTP. To classify paraseptal emphysema, it is necessary to capture location of the emphysema (e.g. near the chest wall or not). According to the results of the present study, it is speculate that the use of larger R leads to implicit encoding of location information in texture histograms.
Although the performance of combined use of LBP and CT density histogram was assessed in both our study and [8] , there were differences in the classification accuracies and optimal parameters between our study and [8] . It is speculated that these differences were caused by the patients cohort and radiation dose of CT. Compared to our study, airflow limitation of COPD smokers was severe in [8] according to results of FEV1. Therefore, when selecting CLE or PSE ROIs in the current study, less emphysematous lesion was included in the ROIs. In addition, the CT radiation dose in the current study was lower than that in the study of [8] . These factors made it difficult to precisely capture texture change caused by emphysema in the current study, and altered the classification accuracies and the optimal parameters.
There are some limitations in the current study. First, because we focused on the texture analysis, noise reduction of CT images was not assessed in this study. Recently, the effectiveness of iterative reconstruction has been shown on low-dose CT [25] [26] . If low-dose CT images obtained by iterative reconstruction were analyzed, classification of emphysema subtypes could be achieved more accurately. Second, only ROI-based classification was performed in the present study. To evaluate lung tissue destruction globally in COPD patients, entire lungs can be analyzed using the same methodology. For future study, we will extend ROI-based classification method for this purpose.
5. Conclusion
The results of this study showed that, on low-dose CT images, texture analysis using LBP, CLBP, and LTP allowed classification of emphysema subtypes. In addition, combined LTP and combined CLBP slightly outperformed combined LBP in classification accuracy.
Acknowledgements
We would like to express the deepest appreication to Sumiaki Matsumoto.
NOTES
*Corresponding author.