Is the Causal Nexus between Agricultural Commodity Futures and Spot Prices Asymmetric? Evidence from India ()
1. Introduction
The agriculture sector is crucial for Indian economy and has a major role in the overall socio-economic framework of India, even though its contribution to the economy has declined over the past 50 years. It generates more than 50 percent of employment opportunities in the country and forms the resource base for a number of agro-based industries and services. It would be more reminiscent to consider agriculture not as a farming activity alone, but as a holistic value chain, which includes cultivation, procurement, warehousing and logistics, pro- cessing and marketing. Since agriculture is the livelihood of the majority of the people in rural areas (around two thirds of people in India are living in rural areas) the demand for many of the industrial products depends on the growth of agriculture sector. Thus, the influence of the agricultural sector on other sectors of the economy through backward and forward integration is enormous.
India is rich with arable land (second largest in the world) and is a major producer and consumer of many agricultural products. However the growth rate of agriculture and allied sectors of the economy are very low compared to other sectors. The annual growth rate of agriculture and allied sector in the GDP is 1.8 percent for the financial year 2012-2013, and the average annual growth of agriculture and allied sector during the Eleventh Five Year Plan period (2007-2012) is 3.6 percent. Since, the majority of the rural population in India is dependent on agricultural and allied activities, slow agricultural growth is a concern for policymakers and “growth with inclusiveness” can be achieved only when agriculture growth rate accelerates. It is evident that growth in agricultural commodities has far reaching ability to impact poverty eradication and rural development. According to World Bank India Country Overview, 2011, “Going forward, it will be essential for India to build a productive, competitive, and diversified agricultural sector and facilitate rural, non-farm entrepreneurship and employment. Encouraging policies that promote competition in agricultural marketing will ensure that farmers receive better prices”. The Indian farmer obtains on an average just one fifth of the price the Indian consumer pays for the same commodity, the difference going into losses, inefficiencies, crop failures and middlemen traders. Even though, the role of government agencies to act as a safeguard and help in stabilizing the market prices, agricultural prices in India continue to be volatile and the production to a great extent dependent on weather conditions.
In this context, the agricultural commodity futures market in India can play a significant role in the price discovery and price risk management. A well developed and effective agricultural commodity futures market facilitates price discovery and thereby, helps in minimising the price risk associated with seasonal variations in the demand and supply of agricultural commodities. Thus, futures markets serve as a mechanism to reduce the price risk associated with commodities by revealing the information about future spot market prices and refers to the use of futures price for pricing spot market transactions. This means that futures price functions as market’s expectations of subsequent spot price. The extent to which futures market performs the price discovery function well can be measured from the temporal causal relationship between futures and spot prices. If the information is reflected first in futures price and subsequently in spot price, futures price should lead spot price, indicating that the futures market performs the price discovery function and it is usually regarded as the leading indicator of judging the efficiency of the futures market. In the literature, a pre-condition for market efficiency is the convergence of both future and spot prices across the market spectrum. It explains how fast one market incorporates information relative to the other, and also indicates the efficiency of their functioning as well as the degree of integration between the two markets.
There have been a number of studies in the literature to examine the temporal causal relationship between agricultural futures and spot prices. These studies assume that the causal relationship between futures and spot markets are symmetrically related in the short run and or in the long run. But, an important issue that has been neglected in the previous studies is to allow for asymmetric nature of information in the investigation of the temporal causal relationship between agricultural commodity futures and spot prices. The impact of negative information (shocks) might be different than the impact of positive information even in a situation in which the magnitude of the shock is the same in absolute terms. In the existing literature, there is no separation between the causal impact of positive and negative shocks. This might be a too restrictive assumption because in many situations, there are potentially asymmetric structures regarding the causal impacts. For example, for a given a unit of negative information and a unit of positive information in the financial markets, people respond more to the former than to the latter. This is because individuals care more robustly about a loss in utility than they do about a gain of equal magnitude. Generally, negative information in the agricultural market generates a much stronger psycho-physiological response than positive information, since market participants are more reactive and conscientious to negative news than they are to positive news. This may lead to asymmetry in the temporal causal relationship between agricultural commodity futures and spot prices. Therefore, in a purely competitive agricultural market, the nature of information (positive or negative) and its asymmetric effect on the causal relationship between spot and futures market is very crucial for the farmers and other market participants for an effective financial risk management through arbitraging and hedging.
The objective of this paper is to investigate the potential asymmetric causal relationship between Indian agricultural futures and spot prices by employing asymmetric causality test introduced by Hatemi-J [1] . Unlike standard causality tests, an asymmetric causality test splits the causal impact of positive shocks from negative shocks. Moreover, the test functions well even if the sample size is small and the data set is not normally distributed. This paper is the first endeavour, to our best knowledge, to investigate the asymmetric causal relationship between agricultural commodity futures prices and spot prices in the Indian context. Since India is either one of the major producer or consumer of many agricultural commodities and its growing global presence as a fast emerging market, understanding the temporal asymmetric causal relationship between the agricultural futures and spot prices is of paramount importance to various stakeholders of commodity markets such as farmers, producers, traders, commission agents, commodity exchange’s participants, regulators and policy makers. The calculation of the dynamic hedge ratio and the effectiveness of hedging are depend on the proper understanding of causal asymmetric relationship. Moreover, the entire exercise associated with forecasting of the spot or futures prices is also dependent on the specification based on proper knowledge about the nature of asymmetric response of the market participants to the shocks. Hence, the results of present study would help in effective hedging and forecasting of agricultural futures and spot prices. The remainder of the paper is organized as follows: Section 2 briefly reviews the literature and Section 3 describes the data used. Section 4 deals with the econometric methodology and Section 5 present the results of the study. Section 6 ends with concluding remarks.
2. A Brief Review of Literature
In a perfectly efficient and frictionless market, futures price should move concurrently with the underlying spot price without any lead or lag in price movements across markets [2] . However, institutional factors such as liquidity, inherent leverage, transaction costs, and other market restrictions, futures market processes information faster than the spot market and may produce an empirical lead-lag relationship between price changes in the two markets [3] . The study of the causal relationship between spot and futures prices is functional to the analysis of the price discovery role futures markets [4] . There are several studies that have been explored the causal relationships between agricultural commodity futures and spot prices.
The seminal work of Garbade and Silber [5] develop a partial equilibrium model which characterises the price movements in cash and futures markets for storable commodities. They study seven commodities and found that all markets are integrated over a month or two, but there were a considerable slippage between cash and futures markets over shorter time intervals. They conclude that, in general, futures markets dominate and lead cash market price changes. Beck [6] examines the long run and short run market efficiency for eight and 24 weeks horizons, with the help of cointegration and error correction model. He concludes that futures markets are sometimes inefficient, but that no market can be found to be always inefficient among cattle, orange juice, hogs, corn, copper, cocoa and soybean. Kellard, Newbold, Rayner and Ennew [7] find that the futures markets for soybeans, live hogs and live cattle are efficient in the long run, but short-run inefficiencies were observed.
Yang, Bessler and David [8] examine the price discovery performance of the futures markets for storable (corn, oats, soybean, wheat, cotton, and pork bellies) and non-storable (hogs, live cattle, feeder cattle) commodities. They use cointegration procedures and vector error correction models (VECM) and find that the storability does not affect the price discovery process, and, for both storable and non-storable commodities, futures markets lead the spot markets. McKenzie and Holt [9] study the long run and short run market efficiency for four commodities using cointegration and GQARCH-M-ECM. The results show that live cattle, hogs, corn and soybean meal futures markets are both efficient and unbiased in the long run, however, some inefficiencies and pricing biases in the form of a dynamic lag structure exist in the short run. Wang and Ke [10] test the efficiency of the futures markets for agricultural commodities and their results suggest a long-term equilibrium relationship between the futures price and cash price for soybeans, and a weak short-term efficiency of the soybean futures market. Zapata, Fortenbery and Armstrong [11] examine the efficiency of sugar futures and report that future markets for sugar leads the cash market in price discovery. A unidirectional causality from future price to spot is revealed.
In India, significant and relevant literature on causal relationships between agricultural commodity futures and spot prices is thin. Kumar and Sunil (2004) investigate the price discovery in six Indian commodity exchanges for five agricultural commodities. They find that the Indian agricultural commodities futures markets are not yet mature and efficient. Iyer and Pillai [12] examine whether futures markets play a dominant role in the price discovery process. This study extends the framework developed by Garbade and Silber [5] , by superimposing a two-regime TVAR model to quantify the price discovery process. The study finds evidence for price discovery process in the futures market in five out of six commodities. However, the rate of convergence of information is slow, particularly in the non-expiration weeks. Ali and Gupta [13] study the efficiency of the futures market for 12 agricultural commodities. The analysis of short-term relationship by Granger causality test indicates that futures markets have stronger ability to predict subsequent spot prices for chickpea, castor seed, soybean and sugar as compared to maize, black lentil and pepper, where bi-directional relationships exist in the short run. Goyari and Jena [14] study the daily spot and futures prices of gold, crude oil and guar seed. The results of the cointegration tests state that both markets are cointegrated for three commodities, suggesting that there is a long term relationship exists between spot and futures markets. Sanjay, Namita and Rajeev [15] examine the short run lead-lag relationship between futures and spot prices. The results of Granger causality test show that there is a bi-directional Granger lead relationships between spot and futures in all agricultural commodities except turmeric in which there is no causality exists. Joseph, Tiwari and Sisodia [16] examine the causal relationship between commodity futures and spot prices for eight commodities by using frequency domain analysis. The results of frequency domain analysis suggest that there is a strong unidirectional relationship from futures to spot in almost all the selected commodities.
It is worth noting that most previous studies ignore the possibility of asymmetric nature of information in the investigation of the temporal causal relationship between agricultural commodity futures and spot prices and the empirical evidence and policy recommendations based on standard causality tests are truly misleading. Hence, in this study, we employ the asymmetric causality tests to investigate the asymmetric causal relationship between Indian agricultural commodity futures prices and spot prices, which has never been used in the Indian context on this topic before.
3. Data
The data sets are price series of futures and spot closing prices of 11 agricultural commodities (i.e. soy oil, chana (chickpea), castor seed, cotton oilcake, mustard seed, soy bean, coriander, turmeric, jeera (cumin seed), pepper and wheat) at a daily frequency, spanning the period from January, 2008 to March, 2014. The price series are collected from the website of National Commodity and Derivatives Exchange Limited (NCDEX) and it is the leading agricultural commodity derivatives exchange in India with agricultural commodity market share of more than 70 percent based on Average Daily Traded Value (ADTV) and more than 80 percent based on open interest. It has more than 700 certified warehouses across India with capacity to handle more than 2.6 million tonnes. The selected commodities are the top actively trading commodities and which constitute on an average 90 percent of the total turnover of NCDEX.
In order to construct the futures price series, the nearby futures contracts are used because these are highly liquid and the most active contracts. Instead of splicing together the nearby futures contracts observations at or near the last trading day, the study employs a different splicing method. First, the nearby futures contract, which is a contract with the nearest active trading delivery month to the day of trading, is specified. Then based on consistent and objective criteria, trading activity is taken as the parameter for splicing the price series. Whenever a contract approaches maturity, the market changes its attention away from the nearby month contract to the next nearby contract before the nearby contract approaches its last trading day. It is important to notice that trading volume and open interest for a particular contract peak three or four weeks prior to the last trading day and start declining. The present method evades using observations near the maturity date of the contracts, which represent weakening and wobbly market interest. The criterion established to identify the shift from the nearby contract observations to the next nearby contract is based on when both the daily trading volume and open interest for the next nearby contract surpass those for the nearby contract; it is considered as the evidence of shifting market’s attention from the nearby contract. At this point we roll-over the series to the next nearby contract.
4. Methodology
Asymmetric Causality Test
The most widely used way of analysing causality is employing Granger [17] causality test, which uses a VAR model to analyse the causality between the variables. However the F test procedure in Granger’s causality test is not valid if the data are nonstationary. Todo and Yamomota [18] introduce another causality test, popularly known as Todo and Yamamoto Granger non-causality test, which can be used in instances of integrated variables and even if there is no cointegration between the variables. But these tests cannot be used to examine the asymmetric relationship between the variables. The methodology followed in this paper is based on the asymmetric causality test suggested by Hatemi-J [1] and this test disengages the potential causal impact of negative shocks from the positive shocks. The future prices and spot prices are denoted by F and S respectively integrated variables and can be defined as:


where
. F0, S0 represents the initial values of F and S, and the ε1, ε2 follows white noise process. We define;

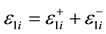
Since positive and negative shocks are defined as
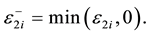
Therefore
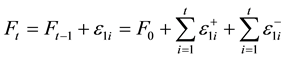
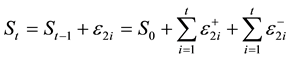
Assuming permanent effect of negative and positive shocks on the underlying variable, the positive and negative shocks of each variable can be defined in a cumulative form as
,
Following Hatemi-J (2012) these positive and negative components are can use for examining the asymmetric causality between S and F.
For examining the asymmetric causality between the positive components of F and S;

is the 2 × 1 vector of variables, α is the 2 × 1 intercept vector and
is the 2 × 1 vector of error terms.
is a 2 × 2 matrix of parameters for lag order r
. Optimum lag order L is defined by using Hatemi-J A (2003, 2008).
The null hypothesis is set as:
H0: the row ω, column k element in Ar equals zero for ![]()
The following denotations are set before defining the Wald test in compact form
(n × T) matrix,
(n × (1 + nL)) matrix
((1 + nL) × 1) matrix, for
,
((1 + nL) × T) matrix, and
(n × T) matrix.
The VAR (L) model define as
![]()
The null hypothesis of non-Granger causality, H0:Cβ = 0 is tested by the following test method
![]()
where β = vec(D), vec = column stacking operator;
= Kronecker product, C = is a p × n(1 + np) indicator matrix with elements, ones for restricted parameters and zeros for the rest of the parameters.
SU is the variance-covariance matrix of the unrestricted VAR model estimated as
![]()
where, m is the number of parameters in each equation of the VAR model. When the assumption of normality is fulfilled, the Wald test statistic above has an asymptotic χ2 distribution with the number of degrees of freedom equal to the number of restrictions to be tested (in this case equal to L).
As first step
with restrictions implied by the null hypothesis of Granger non-causality imposed. Then the bootstrapped data
generated by using estimated coefficients from the regression, the original data and the bootstrapped residuals
. The bootstrapped residuals
are created by T random draws with replacement from the regression’s modified residuals, each with equal probability of 1/T. The mean value of these residuals is zero since it is mean-adjusted by subtracting the mean value of the resulting set of drawn modified residuals from each of the modified residuals in that particular set. These modified residuals are the original residual that are adjusted via leverages to have constant variance. The distribution of the test is generated by bootstrapped simulations of ten thousand times and each time the Wald test is estimated. The bootstrapped critical values of the δ-level of significance
is obtained by taking the δth upper quantile of the bootstrapped Wald test distribution. For testing the null hypothesis of non-Granger causality, the Wald test statistic from the original data is compared with the bootstrapped critical value
. The bootstrap critical values are created for three different significance levels. The bootstrap simulations are executed by using statistical software GAUSS1.
5. Results
The empirical methodology comprises four steps. In the first, we examine the presence of unit roots and existence of cointegration between the spot and futures prices. The results indicate that the study variables are non-sta- tionary and the spot and futures prices are integrated of order one for all the 11 commodities under the study2. Therefore, as the second step, it is imperative to incorporate an unrestricted extra lag in the VAR model in order to account for one unit root according to Toda and Yamamoto [18] . Further, based on the results of multivariate diagnostic tests3, it is evident that the data is not normally distributed and the presence of time-varying volatility. Therefore, it is crucial to employ bootstrap simulation method, as described in the methodology section, in order to attain the accurate inferences. Table 1 provides the results of Toda and Yamamoto [18] Granger non-causality test and results show that there is a unidirectional causality running from futures to spot markets for all the commodities. This implies that futures markets have stronger ability to predict subsequent spot prices and having a dominant role in the price discovery process.
One of the underlying assumptions associated with the potential causal relationship between futures and spot prices is that futures prices influence spot prices but not vice versa. The major argument for the premise that futures prices lead spot prices is that the former respond to new information more quickly than the latter due to lower transaction costs and ease of shorting. In the agricultural market, if new information indicates a rise in prices of commodities, a speculator has the option of either buying futures or spot. However, spot transactions require more initial outlay and possibly time-consuming to implement, while futures transactions could be executed instantly without holding in the physical commodity and with modest up-front cash. Likewise, hedgers who are concerned for the physical commodity and have storage limitations may prefer to buy futures contracts. Hence, both hedgers and speculators would respond to the new information by choosing futures rather than spot
![]()
Table 1. Todo and Yamamoto Granger non-causality test results4.
transactions. Spot prices will react with a lag because spot transactions cannot be executed so quickly.
The third step is to examine the presence of asymmetric causality with the help of asymmetric causality test suggested by Hatemi-J [1] . Table 2 provides the results of asymmetric causality between futures and spot prices. The study finds bidirectional causality between futures and spot prices of castor seeds, RM seeds, coriander and turmeric. However, the presence of causality from spot to futures is only for negative components. For all other commodities under the study, we only find significant causality running from futures to spot prices in both positive and negative components.
The result of asymmetric causality test is appealing because the study find presence of causality running from spot to futures market in case of four commodities namely, castor seeds, RM seeds, coriander and turmeric. But it is not discernible in the results of Toda and Yamamoto [18] Granger non-causality test. Moreover, the presence of asymmetric causality running from spot to futures is evident only in case of negative components of information. This may be because of the asymmetric behaviour of market participants to negative and positive news and is ignored in Toda and Yamamoto [18] Granger non-causality test since they assume symmetrical relationship between spot and futures market. As mentioned earlier, since market participants assign more weight to negative news than that of positive news, it is important to decompose the information content into positive and negative while analysing the causality.
The causality test results for castor seeds, RM seeds, coriander and turmeric are not supporting the well discussed argument that, spot market lags in price discovery due to market constraints like high transaction cost, less liquidity, low leverage etc., We believe this is because of the nature of information content and market’s
![]()
Table 2. Asymmetric causality test results.
reaction to it; asymmetric response. Initially, arbitrageurs will respond to the violation of the cost-of-carry condition and then speculators will modify their expectation of the spot price and react to the difference between expected spot and futures price. Thus, a spot price change may lead to reaction from the all market participants and subsequently cause changes in the futures price. One of the possible reasons may be the potential temporal lead-lag patterns between futures and spot prices dynamically change as market participants filter nature of information relevant to their positions. As a result, decomposing the information content into positive and negative components provide more useful insights into the nature of causal relationships between agricultural spot and futures market.
The above results further motivate us to estimate the asymmetric causality parameter. The basic rationale behind estimating the asymmetric causality parameter is to ascertain the influence of positive and negative information on the asymmetric causal relationship between futures and spot markets. The asymmetric causality parameters for negative shocks are higher than that of positive shocks in all cases except for chana (chickpea) and turmeric. For example, in the case of soy oil, the asymmetric causality parameter from futures to spot market for positive shock is 1.21 and for negative shocks the parameter value is 3.22. More specifically, 1% increases in the futures price of soy oil will causes 1.21% increase in the spot price of soy oil; but 1% decrease in the futures price of soy oil will leads to 3.21% decrease in the spot price of soy oil. The values of significant asymmetric causality parameters for other commodities for negative and positive shocks are given in the following Table 3. The value of asymmetric causality parameter from futures to spot market for positive shocks vary from 0.65 (for pepper) to 4.1 (for chana), while the value of asymmetric causality parameter for negative shocks from futures to spot is lowest for pepper (1.76) and highest for wheat (4.56). The asymmetric causality parameter from spot to futures for negative shocks has highest value for castor seed (1.54) and in all other markets the parameter value is less than 1.
![]()
Table 3. Asymmetric causality parameter values.
6. Conclusions
Agricultural commodity futures exchange provides a centralized marketplace where the farmers and other market participants can discover the prices of agricultural commodities for futures delivery and where risk-averse people can shift commodity price risk to others, who are willing to bear it. Ali and Gupta [19] highlight that the sustainability of agricultural commodity futures markets depends on the transparency and efficiency of its functioning in terms of price discovery, price risk management, flexible contact specification, controlling unfair speculation, commodity delivery system and coverage, infrastructural support, etc. Given the importance of agricultural futures market, we contribute to the literature by analysing the asymmetric casual relationship between spot and futures prices of agricultural commodities in the Indian commodity market by employing the asymmetric causality test of Hatemi-J [1] , which has never been used in the Indian context on this topic before. The Asymmetric response by the investors to positive and negative shocks and its possible effect on the price discovery process is emerging research area in finance. The results of the present study are very useful for the market participants to understand the asymmetric causal impact of positive and negative news on prices of agricultural commodities separately, which would leads towards an effective financial risk management through arbitraging and hedging.
The Toda and Yamamoto [18] Granger non-causality results indicate the presence of unidirectional Granger causality from futures to spot prices for all the commodities. However, the Toda and Yamamoto [18] Granger non-causality test results does not indicate whether the positive and negative changes in one market cause changes in other market price. The asymmetric causality test results indicate that futures to spot market price causalities are significant in all the eleven commodities under study in both positive and negative components. In addition, the results show the presence of causality from spot to futures for coriander, RM seeds, castor seeds and turmeric. For coriander, spot to futures price causality is significant in both positive and negative components, while for RM seeds, castor seeds and turmeric the spot to futures causality is running only in negative components. The values of asymmetric causality parameters of the significant causal relations show that the parameter values are higher in case of futures to spot market causality for negative components (except for chana). This shows that negative shocks coming from the futures markets are having more impact on the spot market’s price than that of the positive shocks. In other words, the market participants are assigning more weight and responding more quickly to negative shocks than the positive shocks. There are several reasons behind the presence of potential asymmetric temporal causal relationship between agricultural futures and spot prices. The heterogeneous expectations from the part of market participants in the agricultural markets may lead to asymmetric response. When a negative shock batters agricultural prices, it may bring uncertainty and pessimism among the market participants and leads to limiting their activity. Hence, such a negative shock may narrow the basis. But, when a positive shock strikes the markets, the same market participants will start to increase their activity due to optimism, but these are mainly subject to supply constraints, leading to asymmetric adjustment. Moreover, positive or negative news related to the existing levels of inventories and related convenience yield may also have asymmetric impacts on futures and spot prices.
In agricultural commodity markets, prices are determined by fundamental supply and demand forces which are undergoing structural change due to the increasing demand from the emerging economies, alternative uses of agricultural commodities for energy production, and supply side constraints. At the same time, trading activities on agricultural commodity derivative markets have also undergone a major change due to the growing presence of financial investors. Institutional aspects like government intervention and other factors like political, regulatory, environmental and economic shocks may also have asymmetric responses to the behaviour of futures and spot prices. The findings in this study have important implications for market participants and policy makers. Testing of asymmetric causality after decomposing the information into positive and negative components would help market participants to predict the price in the respective markets according to the nature of information components. With the help of asymmetric causality parameter, the market participants can ascertain the influence of positive and negative information on the asymmetric causal relationship between futures and spot markets and thus they can design and implement appropriate trading strategies on the basis of nature of the information content.
NOTES
1The software program written in GAUSS to compute the cumulative sums and for running leveraged bootstrap simulations for the causality tests are available upon request.
2Under Toda and Yamamoto [18] test, cointegration between the variables is not a prerequisite for analyzing causality. The results of unit root and cointegration is available upon request to the authors.
3The results of multivariate diagnostic tests are available upon request to the authors.
![]()
4Unit root test and cointegration results will be provided up on request to corresponding author.