Calibration of Nondestructive Assay Instruments: An Application of Linear Regression and Propagation of Variance ()
1. Introduction
“Simple linear regression” refers to fitting a response y as a linear function of a single predictor x [1] . Although calibration of assay methods sometimes requires more than a single predictor x, simple linear regression is often adequate. In fact, assay developers often aim for a simple linear relation between a quick-to-measure response and a single predictor, such as radioactive source strength. Regression fitting is used during calibration and then to apply the assay method, the quick-to measure response is used to predict the source strength (such as grams of nuclear material) of new test items.
This paper illustrates simple linear regression, residual diagnostics, and variance propagation in a form suitable for implementation, intended both for practitioners who calibrate instruments and also as a case study of good applied statistical practice. In particular, much of our experience is in nondestructive assay (NDA) measurements such as neutron and gamma detection which through careful physics-based modeling and calibration can infer grams of source material without touching or sampling from the item [2] . The NDA professional has, however, only a meager set of widely used texts that have partial examples to serve as a common, basic approach for all NDA practitioners [3] -[5] . Within the NDA literature, we are not aware of any examples that fully describe the statistical procedures and illustrate good practice. The classic book by Sher and Untermyer [5] surveys a broad range of NDA techniques. Statistical analysis for assay systems is covered in Chapter 8, where Jaech [5] provides an example of establishing a calibration that is linear in the predictor by the method of weighted least squares (WLS), and shows how to apply it to assay a group of items. A large and important class of NDA applications is that for nuclear safeguards [6] , with the goal to measure and account for special nuclear material to meet international agreements.
Given the ubiquitous nature of linear calibrations, and also because such analysis often provides a stepping off point for more complicated forms of instrument response (such as models that are linear in the parameters but that include transformations of the predictors), it is important for NDA practitioners to understand the standard underlying statistical approach. The treatment in [5] covers a number of salient points but is insufficient in several important ways. The purpose of this article is to extend the analysis in [5] and create a worked example that informs the uncertainty quantification sections of NDA standard guides with respect to good practice. In particular, we:
• Extend the analysis to non-transformed coordinates because this is what most NDA software uses and illustrate how random and systematic error variances are estimated from non-transformed coordinates;
• Evaluate the impact of estimation errors in the variances that are used for weights in WLS;
• Extend the uncertainty treatment by including scaling for the “external” estimate of how well the regression lines represents the calibration data set;
• Present graphical uncertainty bands;
• Examine whether repeat data is consistent with the assigned uncertainties;
• Discuss overall goodness of fit metrics including the use of residual plots, the correlation coefficient, and the chi-squared
per degree of freedom;
• Transform (or invert) the calibration line of regression so that it may be used to perform assays. This provides an example of propagation of variance (POV) based on first order Taylor series expansion. This step is usually not developed in NDA standard guides.
2. Statement of the Measurement Problem
Calibration data for a uranium assay system is given in Table 1. The uncertainty in the mass of each calibration item is negligible compared to other contributions so is ignored here. The uncertainty in the net observed counting rate is defined as the standard deviation. The standard deviation estimates are based on historical experience and are given in [5] without further justification. A model that is linear in the predictor is assumed. If exploratory analysis suggests that a more complicated model is necessary, then in practice least squares fitting is still often used, particularly if the chosen model is linear in its parameters and covering a limited dynamic range of operation is adequate.
After calibration, the system is used to assay three unknown items of the same type as used for calibration. The measured data is listed in Table 2. The purpose of the measurement is to estimate the total amount of 235U present in the three unknown items and to provide a defensible uncertainty on the aggregate amount.
In adapting the problem from [5] we are retaining more significant digits in the rates than can be statistically justified simply to avoid gross rounding errors in our comparison to the treatment in [5] .
As a second problem we assume the calibration data were acquired by taking each calibration item through a complete measurement cycle four times as shown in Table 3. Supposing this is all the information one had, we
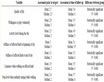
Table 1. Calibration data; counts per second as a function of 235U mass in grams.
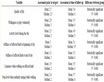
Table 3. Alternate calibration data with four repeat measurements of each calibration item.
perform the calibration and apply it to the same unknown set.
3. Recap of the Well-Known Weighted Least Squares (WLS) Solution
We assume there is a linear relation between the predictor variable,
, and the measured quantity,
, so
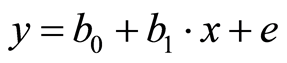
with
. The error term e is assumed to be randomly distributed around a mean value of zero.
It is assumed that experimental uncertainties exist in the
values but that the
values are known exactly. The calibration data consists of a set of
measured points
, with standard deviation
denoted
when the meaning is clear from context, which in problem 1 we assume known, following the treatment in [5] . Because we are fitting y to x at this stage, the next stage will solve for unknown x values in terms of observed y values. Alternatively [7] , one could directly fit x as a function of y, but this involves “errors in predictors” so we do not consider that approach here.
We introduce the notation:
.
The WLS solution [3] [4] [8] may be written, using the “hat” notation to denote estimates based on the data:
, 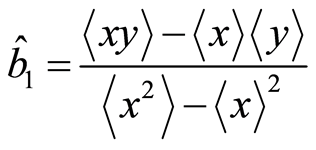
The variance and covariance estimators are given by the following expressions:
,
, 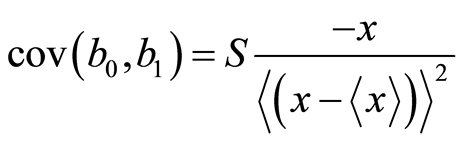
where
is the external variance of the calibration set defined as the weighted sum of the deviations between the
values and the predicted values
; in this case, for
degrees of freedom,
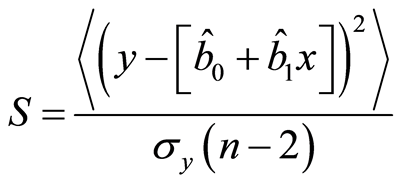
For large data sets for which the assumed standard deviations i are approximately correct,
will be close to unity. In the treatment by [5] ,
is implicitly set to unity. However, using
as a separate parameter to judge the overall quality of the fit is recommended, as we do in the numerical example below. Also, as in the case of the present worked example, often
is not “large.”
The WLS expressions can be understood by transforming the measurement equation y = b0 + b1x + e to one for which the error variances are all identical. That is, ordinary least squares is a special case of WLS and the WLS expressions are the same as the OLS expressions on transformed variables. Specifically, to motivate the WLS expressions using matrix notation, write
with the X matrix being a matrix with two columns and the covariance matrix of e denoted. The first column of X is a column of 1’s and the second column is the values
. Then the OLS solution is
and the WLS is the same as the OLS but with the factor
multiplied on both sides of
to transform the unequal-variance case to the equal variance case for which OLS is appropriate. The result is
. In the simple case considered here,
is a diagonal matrix with entries
along the diagonal.
The equations can be simplified by a translation of variables to center the coordinate system about the point
where
and
take on numerically exact values equal to
and
respectively. Thus writing (and through context avoiding any possible confusion with the X-matrix):
, 
we have
, 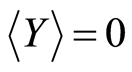
and the WLS expressions simplify in the sense that the covariance term between coefficient estimates vanishes because the estimated intercept is always zero. Reference [5] elects to work exclusively in terms of this translated coordinate system. However, when calibration parameters are to be entered by an operator into NDA analysis software the normal convention is to work in the original data space. In what follows, therefore, we work with the non-translated coordinates.
The WLS line passes through the weighted centroid of the calibration data so that the relation between
and
can also be written as:

If we define
, 
and

then we can write the relation between
and
as:
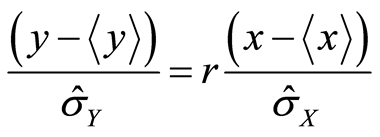
The quantity
is known as the coefficient of correlation. Numerically it is bounded by the interval 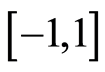
having the same sign as
and hence the same sign as the gradient. It is a measure of the strength of the correlation between the variables
and
and is often also reported along with the extracted coefficients, although [5] does not do so. More generally, r2 measures the fraction of variance of y that is explained by the linear function of x. Values of r2 near 1 such as 0.99 indicate that there is very little room for improved calibration by using some more complicated function of x, such as a polynomial in x. We caution that if many different functional forms are evaluated, then artificially high
can be obtained, so there must be some adjustment for “data mining” [9] [10] . However, if the simple linear relation was chosen prior to data collection, and if
is close to 1, then there is little to be gained by examining other possible functional relations between y and x.
4. Calibration
The calibration step can be expressed by the relationship:

where
is the certified 235U mass, s is the observed net counting rate, and the error term is assumed to be random with zero mean value. The model parameters
and
are the fitted calibration coefficient estimated in our example by the technique of WLS as just described. Recall that one could instead directly fit x as a function of y, but this involves “errors in predictors” so we do not consider that approach here [7] [11] .
5. Application
To use the calibration line to perform an assay we invert the relation as:
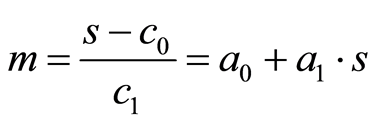
where we have now introduced the assay “calibration” coefficients
and
so that the relation is in the usual form required by typical NDA software. That is to say, a user is prompted to enter the coefficients
and
(not
and
) along with the corresponding uncertainty information. Upon substitution and after applying the standard rules for propagation of variance (POV) we arrive at:
,
,
, 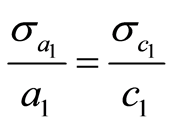

The expression for the covariance term
is the expectation value of the first order expansion, written in terms of statistical deviation
about the mean, of the product
.
It is common in nuclear materials accounting to need to assign a total mass and uncertainty to a collection of
items. Each item has an individual assay measurement
along with associated standard deviation estimate
, where the index
runs from 1 to
(and for an individual assay
). The estimate for the total mass present in the collection is therefore:

Propagating variances yields:

which we can re-express more concisely as:

with
being the sum of the variances of the individual rates calculated, assuming there is no additional connection among the N measurements other than the shared calibration parameter estimates. That is, each of the measurements is assumed to be independently determined. For this assumption to be valid requires, for example, that the collection of measurements for the items under consideration do not share a common background count subtraction. In the case of a gamma-ray spectroscopic assay the intensity under the full energy peak is determined from the counts in the pulse-height spectrum itself on either side of the peak and so this assumption is met, resulting in:
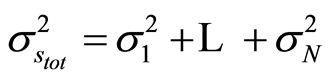
The first three terms in the result for
, those enclosed in the square bracket, would be zero if the calibration coefficients were known perfectly. Collectively these three terms therefore represent the systematic uncertainty, which is specific to the particular collection of items because of the
factor in the second term. The fourth term in the result for
is the variance arising from the uncertainty in the observed counting rates, which in the case of a nuclear counting experiment may be dominated by the Poisson nature of the detection of particles emitted by the radioactive decay process. In such cases the uncertainty in the counting rate may be approximated for each item even when only a single repeat count has been taken, even if a Poisson-distributed background count is subtracted from a Poisson-distributed gross count to calculate the net count.
Perhaps surprisingly, note that some of the pairwise covariances cov(mi,mj) can be negative because when the true regression line is overestimated in one region of the data, it tends to be underestimated in other regions, as we illustrate in Section 6.
6. Numerical Examples
6.1. Example 1 Using Table 1 Data
Applying WLS to the Table 1 calibration data, we obtain
,
, S = 6.28, r2 = 0.998, and
. Applying the POV results for
we get the results in Figure 1 for i
= 1 and j = 2 to 30 in equally simulated test data ranging from the minimum of x (1) to the maximum of x (20) in the training data in Table 1. All analyses, plots, and simulations were performed in the statistical programming language R [12] . For reader convenience, the Appendix lists the R source code to duplicate and extend our analyses—for instance, to create other visualizations of the data or to perform additional calculations. However, any available software including Microsoft ® Excel ™ is adequate for these simple linear regression analyses.
Notice in Figure 1 that
can be negative, which is contrary to the typical situation with systematic errors leading to positive covariance. The reason
can be negative is illustrated in Figure 2.
Figure 2 uses the original 6-point (x,y) training data pairs from Table 1 and a second set of 6 (x, ysimulated) where ysimulated = y + ey with ey drawn from a Normal distribution with zero mean, that is ey~ N(0, σy) with standard deviation σy given in Table 1. Figure 2 illustrates that a fitted line that lies above the “true” line for large x values will tend to lie below the “true” line for small x values (“true” is in quotes because in practice one never knows the true relation between y and x, but this paper assumes the true relation is exactly linear). This means that distantly-spaced x values tend to have oppositely-signed estimation errors, and so
can be negative. In our experience, nuclear safeguards metrology almost never reports negative values of 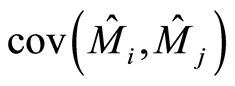
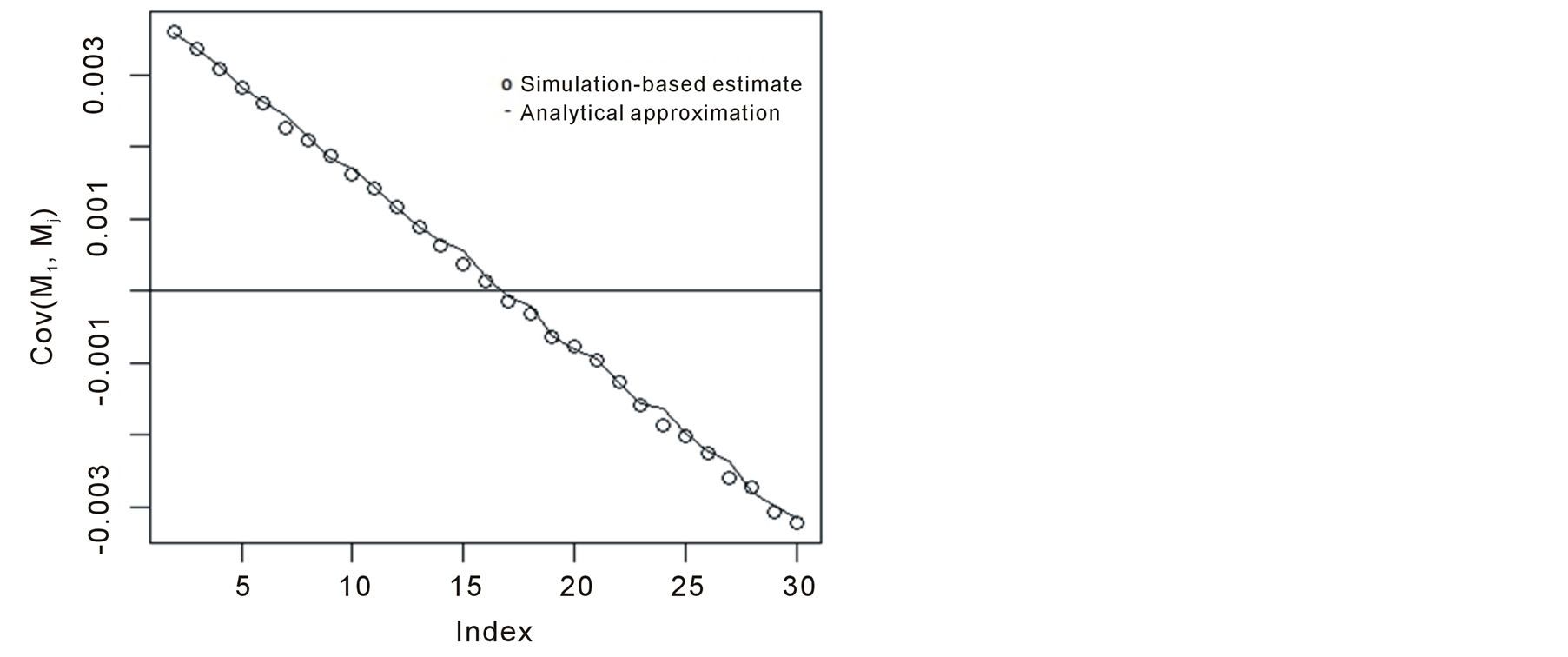
Figure 1. Predicted cov(M1,M2) and observed cov(M1,M2) in 105 simulations.
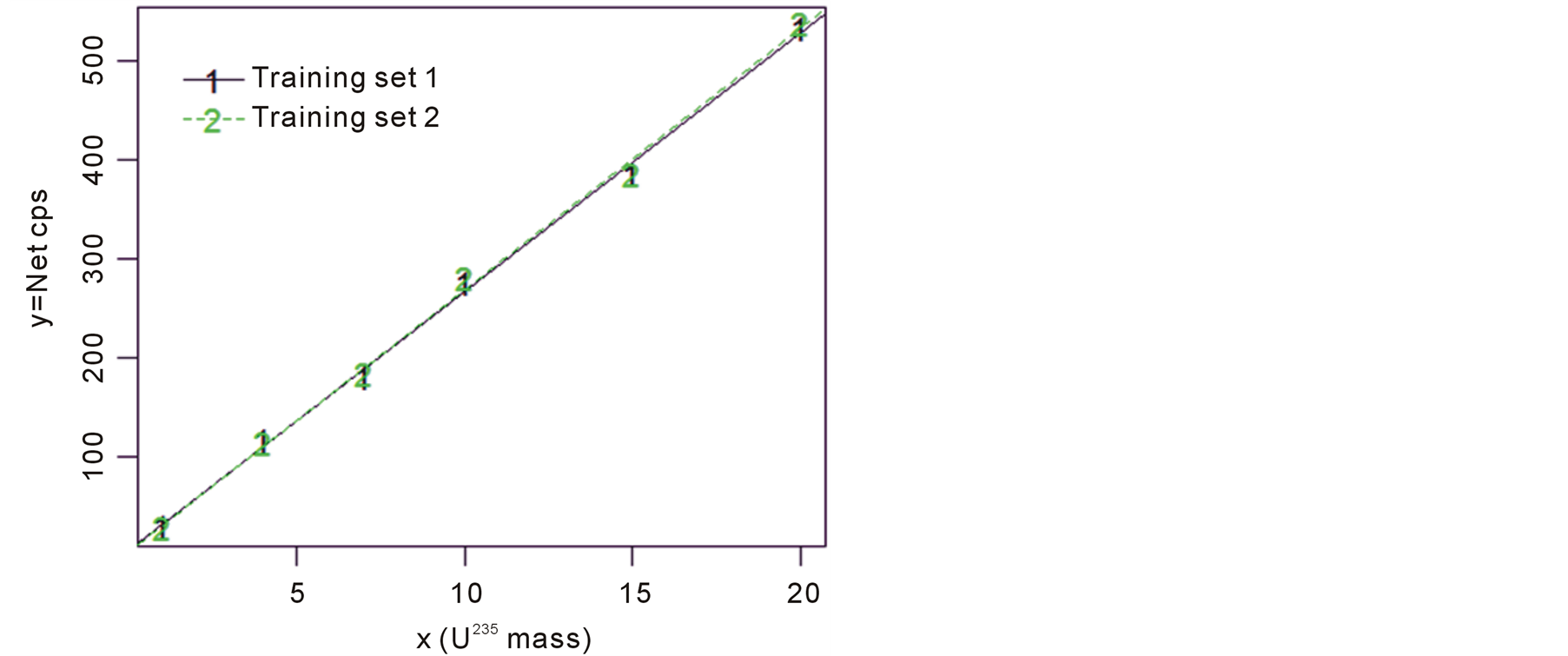
Figure 2. The training set data in Table 1 and a second simulated training set, with the fitted lines to both. Estimation error in the fitted line leads to positive cov(M1,M2) for M1 and M2 that are close in value and to negative cov(M1,M2) for M1 and M2 that are distant in value.
because safeguards tends to use measurement control data rather than calibration data to estimate random and systematic error variances [2] [5] [9] [13] . However, it is helpful to recognize that calibration data can indeed lead to negative estimates of
as seen in Figure 1.
6.2. Example 2 Using Table 3 Data
Next we repeat the previous example but use Table 3 to estimate the standard deviation of y, instead of using the known values of
as used in Example 1. The resulting estimates (the sample standard deviations of each of the four repeated measurements as given in Table 3) are 3.23, 4.17, 14.54, 2.19, 19.60, and 8.01 for
, respectively. The resulting WLS estimates are
,
, and
, S = 0.89, and r2= 0.999. So, although
and
did not change much from the previous example,
did change considerably. The estimates
and
did not change much from the previous example because the points all lie close to a line (Figure 2), so changing the weights has little impact. In other examples, changing the weights can have more impact.
6.3. The Three Test Cases in Table 2 with Variance Propagaion
The three test cases in Table 2 are estimated as 6.47, 2.90, and 13.23 using the first estimate of
and
from Example 1 and as 6.29, 2.81, and 12.87 using the second estimate of
and
from Example 2. Then, applying our approximate result for
from Section 5, we predict a standard deviation of 0.14 for the aggregate of the three items (22.60 using the first estimate of
and
and 21.97 using the second estimate of
and
). To test the quality of our approximate result for
we simulated 105 realizations of the calibration data, repeated the WLS fit, calculated the sum of the three predicted masses, and obtained a standard deviation across the 105 realizations of 0.14 (repeatable across sets of 105 realizations to 0.14), indicating excellent agreement with the predicted standard deviation of 0.14.
6.4. Impact of Estimation Error in the Weights in WLS
Example 1 assumed that that true
values are known, which implies that the exact weights
are known.
Example 2 assumed that the sample standard deviations of the four items were the true
values as a consistency check (see Section 6.5.3 below). In practice, in most situations, the variances will be estimated using a few repeats per item, so uncertainty in the estimated weights should be considered.
In Example 2 with Table 3 data, one might question whether four repeated measurements of each standard is sufficient to obtain reliable estimates of the standard deviation; and, in general, WLS is vulnerable to performance degradation when the weights are not reliably estimated [14] -[16] . Reference [15] showed that estimating weights using the reciprocal of the sample variances is inefficient compared to using a preliminary fit of the regression function as an intermediate step to estimating the weights. But, regardless of the method used to estimate the weights for WLS, the standard deviation of the estimated model parameters is larger than those predicted on the basis of assuming the true weights are known, unless the sample size is quite large, more than 10 per standard. Reference [16] suggested a bootstrap simulation strategy to address the impact of uncertainty in the weights for the purpose of obtaining more reliable confidence statements. Reference [16] gave guidelines that the estimated standard deviation of the estimated slope
and estimated
are each approximately 20% or more too small for fewer than 10 repeats per standard. So, the effect of estimation error in the weights cannot be ignored if in our context we do not simply assume the exact weights
are known.
Fortunately, it is simple by simulation to include the impact of estimation error in the weights (Appendix). Figure 3 plots the root mean squared estimation error (RMSE) in the estimated intercept and slope for n = 2, 3,4,5, 10, and 20 assuming the weights are known, or using the sample variances to estimate
, or inappropriately using the actual repeated data in Table 3 using unweighted least squares. This third option of using the raw data in unweighted least squares is not advised because we know that the variance is not constant across the standards. However, its RMSE performance is still of interest. Notice that for
, all three methods have approximately the same RMSE. But, for
, estimation error in the weights cannot be ignored.
Recall from Section 6.3 that to test the quality of our approximate result for
we simulated 105 realizations of the calibration data, repeated the WLS fit, calculated the sum of the three predicted masses, and obtained a standard deviation across the 105 realizations of 0.14, assuming the weights
are known exactly.
The standard deviation of 0.14 agreed with our approximate variance propagation result of 0.14. However, if we include the impact of estimation error in the weights, the observed standard deviation of the sum of the three masses is 0.18, 0.15, and 0.14, for n = 4, 10, and 25, respectively. So, in this context, again there is close agreement between our approximate result that assumes the variances are known for
. But, for n = 4 we should not use the 0.14 estimate if we must estimate the variances to be used in WLS. Instead, for small n such as n = 4,

Figure 3. The root mean squared error (RMSE) in estimating the intercept
and the slope
assuming the weights are estimated or known exactly in WLS. Also, the RMSE for unweighted LS is plotted.
we rely on simulation to find that the standard deviation of the sum of the three masses is 0.18, which is substantially larger than 0.14.
6.5. Goodness of Fit Testing
No regression model can be blindly accepted without some assessment of goodness of fit (GOF). There are many GOF options and here we describe three that are in common use.
6.5.1. Cook’s Distance to Measure Influence
Cooks’ distance is used to gauge whether any particular calibration data pair (x, y) has unjustified large influence on the values of
and
[1] . Large influence points tend to be those xi with high leverage, meaning that xi is far from the middle x values in the calibration data. This data does not have any calibration data with large influence on the values of
and
.
6.5.2. Residuals versus Fitted Values Plot
Another GOF option is shown in Figure 4, a plot of the scaled residuals
versus the predicted y values, calculated as
. Any pattern in this type of plot indicates a lack of fit to the assumed simple linear regression. Interestingly, Figure 4 shows a see-saw pattern, with alternating signs. Such a pattern raises concern and so a statistical test called a “crossings” test is included in some quality control programs. Here, with only 6 calibration pairs, we will not fail this GOF test, but it does raise concern. It is possible that the dataset chosen by [5] is synthetic; however, because the results of this analysis are not mission critical, no additional investigation is necessary in this case. Another recommended GOF plot is a normal probability plot of the scaled residuals to check for approximate normality of the scaled residuals [1] . It should be noted however that although it is common to assume the residuals e are normally distributed, WLS does not rely on this assumption. Nevertheless, in most applications, it is of interest to evaluate whether the scaled residuals have approximately a normal distribution, because this is informative about how the detector is operating and, for example, whether there is operator bias.
6.5.3. Consistency Checks
Another GOF test available here is based on the scaled variance of the residuals,
, which should be nearly
Figure 4. Scaled residuals versus predicted y values.
equal to one. Formally, the quantity S is distributed as a scaled
random variable. So the value of S = 6.28 for the first values of
and
is large, but not extremely so, because
. The value S =
0.88 for the second values of values of
and
is quite close to 1. Another consistency check compares the estimated total source strength in the three test items using the first values of
and
to the estimated total source strength using the second values of
and
. Recall that the total source strength is estimated at 22.60 (in grams) using the first estimate of
and
and estimated at 21.97 using the second estimate of
and
. The difference 22.60 – 21.97 = 0.63 is much larger than twice the estimated standard deviation of estimated total (
is predicted to be 0.15 by our approximate result and observed to the 0.14 in 105 simulations, which is repeatable across sets of 105 simulations to values that round to 0.14). Therefore, there is some indication of disagreement between our assumed
values from [5] and those calculated from the four repeat measurements in Table 3. One might question whether four repeated measurements of each standard is sufficient to obtain reliable estimates of the standard deviation, and in general, WLS is vulnerable to performance degradation when the weights are not reliably estimated [14] . Because four repeats is not many and because most of the GOF tests indicate reasonable fit, we are satisfied with simple linear regression and with either the first or the second estimate of
and
.
Because the GOF tests suggest that the simple linear regression is adequate, we show in Figure 4 a plot of point-wise approximate 95% confidence intervals around the fitted line from the calibration data. These pointwise confidence intervals are calculated at a value x using the well-known result
, where X is a matrix with 6 rows and 2 columns (column 1 is a vector of 1’s and column 2 is the 6 x values in the calibration data) as in Section 3 [1] [5] . The 95% point-wise confidence intervals in Figure 5 are at the predicted y value
. Simultaneous confidence intervals that have 95% probability of simultaneously including all true y values are somewhat wider [17] .
7. Discussion
In the NDA of special nuclear materials it is common to encounter linear calibrations based on weighted least squares. This article revisited a “text book” example to describe simple linear regression applied to calibration data and variance propagation for the inversion step used to infer the source strength of unknown test items. In evaluating the mass of a collection of test items, the partition between systematic (calibration) and random (assay) variance is important and was clarified and illustrated. Separate reporting of these distinct contributions is not always done by the NDA community. Indeed, many databases in use in the NDA community do not have a

Figure 5. Point-wise approximate 95% confidence intervals around the fitted line from the calibration data.
convenient means to record such information, although it is strongly recommended and may be of great practical importance—for instance in reconciling shipper/receiver differences in nuclear materials accounting or in using a collection of measurement items as a quality control working standard.
Materials control and accounting systems for nuclear safeguards typically estimate random and systematic error variances from either the internals of the measurement process, calibration data (as presented here), measurement control data, or measurement comparison data [2] [5] [13] . We are not aware of published studies showing how such variance estimates vary across the four data sources, but it is usually assumed that measurement comparison data (that provides for inter-comparison of different measurement techniques), are necessary in order to monitor for model departure effects that the three other data sources are unlikely to detect unless experiments are designed and conducted with field conditions in mind.
Although we have not discussed experimental design one can assess whether six standards is adequate by considering the covariance matrix of the estimators,
, whose diagonal entries (the variance of
and of
) can be reduced by spreading out the x values and including more x values.Whether four repeats of each calibration item is enough depends on the magnitude of the true variance
. Many practitioners consider six repeats to be a minimum, as a general rule of thumb, before the sample standard deviation becomes a reliable estimator of the true standard deviation
. To put this in context, the coverage factors for Student’s t-distribution with 5 degrees of freedom are about 1.11 and 2.57 for 68.27% and 95% confidence levels respectively (compared with multipliers of about 1.00 and 1.96 in the limit that the number of degrees of freedom becomes large) [1] . When the number of samples is small one must accept that the uncertainty in the estimates of
will be large and one cannot address the likelihood of rare events in the tail of the distribution. That is, identification and rejection of all but gross outliers is not possible, because with 9 points of fewer the spread is always covered by ±3-sigma. Also, recall that Section 6.4 illustrated that if
, then simulation is necessary to estimate the standard deviation of the sum of source strengths of the three test items.
The use of linear fitting can also form an important aspect of NDA methods in a more subtle way. For instance, the step change in the transmitted intensity of a Bremsstrahlung beam across the K-shell absorption edge is used to the assay uranium concentration of aqueous solution samples. One way to determine the magnitude of the step is to linearly extrapolate a double logarithm of the reciprocal transmission from below, and also to linearly extrapolate from above, with both extrapolations being made to the channel in the spectrum corresponding to the K-edge energy, where the energy calibration is itself also based on a linear relation in terms of channel number. So in this case, which we will not discuss further here, extraction of the predictor variable and placing realistic confidence limits on it involves combining three separate linear dependences. The discussion presented here provides the basis for analyzing this more complicated case and underlies the need to establish good consistent practice among NDA professionals.
We note that if a stable instrument is used for a long sequence of assays on many items of similar type using the same calibration
then the fractional random uncertainty will become small and the group uncertainty will be dominated by systematic uncertainty contributions. In such cases the assumption that the calibration items are known perfectly (perhaps reasonably so for an individual assay) may need to be checked to confirm it remains fit for purpose.
A complementary generalized treatment that admits uncertainties in both x and y has been presented elsewhere [11] . Under this scheme the approach described here for transforming between calibration and assay axes can be avoided because the calibration can effectively be performed as a regression of mass (or source strength) on counting rate directly, with the uncertainties in the rates being propagated into the coefficients in the form they are required for assay. This approach is straightforward to implement but is not yet in common use within the NDA community; this is why we focused on the traditional approach here.
The case of a proportionate response, where the line is known for physical reasons to pass through the origin,
,
, can also be treated using simple statistical concepts [18] .
And, in this case, neglecting correlation in the calibration data can result in misleading conclusions. This is well illustrated by the efficiency calibration of gamma-ray spectrometers that often makes use of nuclides that emit more than one line. In such cases the gamma-ray line intensities are correlated being linked to the same activity certification. An example of how to treat polynomial calibrations with correlated input data of this sort is provided by Henry et al. [19] .
8. Conclusion
The numerical and statistical procedures used to calibrate and interpret the data collected from assay instruments are fundamental to materials accountancy measurements for nuclear safeguards. We have pointed out that there is a lack of explanatory examples in the non-destructive assay literature. Therefore, we extended the treatment of a problem originally posed by [5] to clarify the traditional approach and provided a framework for standard non-destructive assay guides to build on. The treatment of total measurement uncertainties in non-destructive assay measurements presents many more challenges than we have covered in the present discussion. We anticipate increased attention will be given to this field of study in the near future, commensurate with both the importance of achieving high quality assays and the opportunity to improve the state of non-destructive assay practice.
Appendix. Example R Code
x = c(1,4,7,10,15,20)
y = c(28.533,116.108,180.715,275.540,386.488,534.640)
sigma.y = c(2.03,2.42,2.75,3.33,4.16,5.59)
yvar.est = c(10.45209,17.40249,211.5402,4.79046,384.0128,64.20487)
xmat = cbind(rep(1,6),x)
tempcov1 = solve(t(xmat) %*% diag(1/sigma.y^2) %*% xmat)
tempcov2 = solve(t(xmat) %*% diag(1/yvar.est) %*% xmat)
fit0 = solve(t(xmat) %*% diag(1/sigma.y^2) %*% xmat) %*% t(xmat) %*% diag(1/sigma.y^2) %*% y fit1 = lm(y ~ x,weights=1/sigma.y^2) # using lm() function in R gives same results as in fit0 ytrue = predict(fit1) # ytrue is used below in simulation xt = x/sigma.y yt = y/sigma.y
# alternate: lm(yt ~ xt)
# Example simulation nsim = 10^5; xtrain = x; ntest = 3; xuse = xtrain ytest = c(174.19,80.49,351.08) # Table 2
xest = matrix(0,nrow=nsim,ncol=ntest)
coef.est = matrix(0,nrow=nsim,ncol=2)
for(isim in 1:nsim) {
ymeas = ytrue + sigma.y*rnorm(length(ytrue))
temp1 = lm(ymeas ~ xuse,weights=1/sigma.y^2)
xest[isim,] = (ytest-temp1$coef[1])/temp1$coef[2]
coef.est[isim,] = temp1$coef
}
temp2 <- apply(xest,1,sum)
var(temp2)^.5
[1] 0.14 # agrees closely with approximate result σmtot2 = 0.15
# var(coef.est) also agrees with known results
## Impact of estimation error in the weights for WLS nsim = 10^5; ntest = 3 n = 4; ytest = c(174.19,80.49,351.08)
fit1 = lm(y ~ xtrain)
ytrue = fit1$coef[1] + fit1$coef[2]*xtrain xest = matrix(0,nrow=nsim,ncol=ntest)
coef.est = matrix(0,nrow=nsim,ncol=2)
for(isim in 1:nsim) {
ymeas = ytrue + sigma.y*rnorm(length(ytrue))
temp = rep(sigma.y,each=n)*rnorm(6*n)
temp.mat = matrix(temp,ncol=n,byrow=T)
sigma.y.est = apply(temp.mat,1,var)
junk = lm(ymeas ~ x,weights=1/sigma.y.est) # note
#junk = lm(ymeas ~ x, ,weights=1/sigma.y.est) # not used here
xest[isim,] = (ytest-junk$coef[1])/junk$coef[2]
coef.est[isim,] = junk$coef
}
temp = apply(xest,1,sum)
var(temp)^.5