Revolutionizing Education: Exploring the Potential of AI-Enabled Brain-Based Learning for Enhanced Cognitive Development ()

1. Introduction
Education, as a fundamental tenet of society, plays a crucial role in shaping individuals’ minds and promoting societal advancement. With the advancement of the digital era, the integration of cutting-edge technologies into education is undergoing significant changes. One such technology with enormous potential is artificial intelligence (AI) (Bada & Jita, 2022) [1] . AI technologies have made significant advancements in recent years and found applications in various fields, including education. By leveraging AI’s capabilities in data analysis, personalization, and adaptive learning, educational practices can be improved (Kim, Park, & Lee, 2016) [2] .
Brain-based learning is a popular educational strategy that focuses on understanding how the brain acquires and processes knowledge (Ramakrishnan, 2018) [3] . It emphasizes the importance of aligning instructional strategies with the natural functioning of the brain. According to Ramakrishnan (2018) [3] , brain-based learning strategies provide valuable insights for improving learning environments and enhancing student achievement.
AI-enabled brain-based learning, for instance, allows educators to personalize and optimize learning environments based on each student’s unique needs and learning preferences (Wang & Hodgers, 2021) [4] . However, the implementation of AI-enabled brain-based learning approaches also comes with challenges and ethical considerations. Addressing issues such as algorithmic biases, data privacy, and the responsible use of AI is crucial (McCall, 2020) [5] . The integration of AI technologies should be guided by ethical considerations to ensure inclusive and equitable learning opportunities (Varghese, 2021) [6] . The primary objective of this study is to explore the potential of AI-powered brain-based learning techniques in enhancing cognitive development. It involves a comprehensive analysis of empirical studies and research findings to assess the impact of AI on student engagement, knowledge retention, and critical thinking abilities.
Additionally, this paper examines real-world examples and best practices from academic institutions that have successfully integrated AI and brain-based learning concepts. By examining these implementations, valuable insights into effective tactics and strategies for leveraging AI in education can be gained.
2. Methodology
This qualitative study investigates the potential of AI-enabled brain-based learning to enhance cognitive development in the field of education. The study employs a literature review approach, examining published journal articles specifically focused on the utilization of AI and brain-based learning in educational settings. Through a thorough evaluation of these articles, the study aims to gain insights into the effectiveness and implications of AI-enabled brain-based learning for cognitive development in education.
2.1. Literature Search Strategy
A comprehensive search strategy was employed to identify relevant scholarly articles for this study. Electronic databases such as PubMed, Google Scholar, ERIC, and JSTOR were utilized, incorporating a combination of keywords and phrases related to the study topic. These keywords included “brain-based learning,” “AI technology,” “cognitive development,” and “tailored education.” The search was limited to peer-reviewed journal articles published within the last ten years to ensure inclusion of recent research and advancements. By employing this rigorous search strategy, the study aimed to gather up-to-date and relevant literature in the field.
2.2. Inclusion and Exclusion Criteria
Articles were chosen based on their relevance to the research topic in order to better understand the potential of AI-enabled brain-based learning for improved cognitive development. Articles focusing on cognitive development outcomes, applications of AI technology in education, brain-based learning strategies, and their integration were eligible for inclusion. Studies including case studies, conceptual models, theoretical frameworks, and empirical data were given consideration.
Articles were excluded if they were not written in English or did not provide sufficient information about artificial intelligence (AI) and brain-based learning in the context of education. Articles primarily addressing topics outside the purview of the research topic, such as AI applications in medicine or non-educational contexts, were also excluded.
2.3. Data Extraction and Analysis
Data extraction and analysis were systematically conducted on the selected articles. The authors, publication year, research goals, methodology, major findings, and conclusions of each article were extracted along with other relevant information. The extracted data were organized and synthesized to identify common themes, trends, and patterns related to the potential of AI-enabled brain-based learning for improved cognitive development.
A qualitative synthesis of the literature was conducted to gain insights into the key ideas, theoretical foundations, and empirical evidence supporting the integration of AI and brain-based learning in educational contexts. This process involved analyzing and synthesizing relevant studies to identify the advantages, implications, challenges, and factors associated with AI-enabled brain-based learning. A critical review of the literature was also undertaken to ensure a comprehensive understanding of the topic. By employing this approach, the study aims to provide a comprehensive analysis of the current state of AI-enabled brain-based learning in education.
2.4. Quality Assessment
A quality assessment was conducted to ensure the reliability and credibility of the selected articles. The research rigor, methodology, theoretical underpinnings, and applicability of the findings to the research topic were critically evaluated. The articles included in the quality assessment were those that provided solid support and made scholarly contributions to the field of AI-enabled brain-based learning.
2.5. Ethical Considerations
An ethical review was not necessary for this research as it involved a review of published journal articles. However, ethical standards were upheld by ensuring accurate citation and adherence to plagiarism guidelines. Proper citation and acknowledgement of the authors and sources of the reviewed articles were done to honor their original work and uphold intellectual property rights.
2.6. Limitations
This study’s methodology relied solely on a review of published journal articles, limiting the depth and breadth of available information. By focusing on published articles, the study may have missed important details and alternative viewpoints that could have been found in conference papers or unpublished research.
The lack of primary data collection is another limitation. This research relied on the methodologies and interpretations presented in the selected journal articles and did not collect firsthand information from educators, students, or other stakeholders. This constraint makes it difficult to directly evaluate AI-enabled brain-based learning or capture nuanced insights that may only be revealed through close interaction with participants.
The literature review process itself introduces the potential for bias. The selection of articles for inclusion in this study is based on arbitrary decisions regarding inclusion criteria and interpretation. Despite efforts to remain objective and comprehensive, there is a possibility that some relevant articles may have been unintentionally overlooked or omitted, potentially impacting the breadth and depth of the research findings.
Time constraints may have influenced the literature review process due to the extensive body of literature on AI-enabled brain-based learning. As a result, there may be research gaps resulting from the exclusion of certain pertinent studies.
The lack of direct empirical evidence limits the ability to validate the results or draw firm conclusions. While the selected journal articles may cite empirical research, the study’s capacity to directly assess the efficacy or evaluate the outcomes of AI-enabled brain-based learning in educational settings is constrained by the absence of primary data collection.
Furthermore, the generalizability of the findings may be limited. This study primarily focuses on the findings and recommendations presented in the selected journal articles, which may have been conducted with specific populations, contexts, or technological frameworks. Caution should be exercised when applying the research findings to diverse student populations, varied educational settings, or other AI technologies.
Finally, this study does not explicitly address the ethical issues raised in the reviewed articles. When considering the implementation of AI-enabled brain-based learning in educational contexts, ethical considerations such as privacy concerns, data security, and potential impacts on student well-being should be taken into account. Future research should thoroughly investigate these ethical issues to ensure the ethical and responsible use of AI technologies.
3. Principles and Theoretical Foundations of Brain-Based Learning
Brain-based learning is an educational strategy that recognizes the central role of the brain in the learning process and aims to align teaching strategies with its natural functioning (Ramakrishnan, 2018) [3] . It is based on the understanding that the brain is an active processor of information, creating knowledge through various cognitive processes.
Jayalakshmi Ramakrishnan (2018) [3] highlights several brain-based learning principles that have been shown to improve learning outcomes. First, creating a positive and supportive learning environment is crucial in engaging students’ emotions and fostering optimal learning. By promoting a positive classroom culture, teachers can enhance student motivation, reduce stress levels, and encourage an open and collaborative learning environment.
Second, brain-based learning emphasizes the importance of multisensory experiences in the learning process. Studies in neuroscience have shown that engaging multiple senses enhances memory and learning. Educators can incorporate visual, auditory, kinesthetic, and tactile elements into instruction to create richer learning experiences that cater to different learning styles and preferences.
Active learning is another key principle in brain-based learning. By involving students in practical activities, problem-solving exercises, and discussions, active learning promotes student engagement and participation. Such approaches encourage students to actively construct knowledge, deepen understanding, and develop critical thinking skills.
Furthermore, brain-based learning recognizes the importance of reflection and application. Through reflection, students can consolidate their learning, make connections to prior knowledge, and develop metacognitive thinking skills. By applying knowledge in real-world contexts, students can better transfer their learning to new situations, fostering deeper comprehension and long-term memory.
Empirical research has validated these brain-based learning principles, showing that they enhance student engagement, motivation, and knowledge retention (Ramakrishnan, 2018) [3] . By aligning instructional practices with the brain’s natural learning processes, educators can create optimal learning environments that enhance students’ cognitive development and academic achievement.
4. The Current Landscape of AI Technologies in Education
Artificial Intelligence in Education (AIED) has rapidly integrated into educational practices, offering extensive prospects for teachers and students. Its applications in education encompass a wide array of functions, including streamlining administrative tasks like timetabling and resource allocation, as well as enhancing personalized teaching and learning experiences. From managing logistical aspects to tailoring educational approaches, AI has become an influential tool in the field of education (Adams et al., 2023) [7] . One notable application of AI in education is the creation of a museum learning model using brain-based instruction (Kim et al., 2016) [2] . This model utilizes AI technologies to create dynamic and personalized museum experiences that are immersive and interactive for learners. Through AI, learners can explore exhibits, receive personalized information, and engage with virtual artifacts, thereby enhancing their cognitive development and understanding of the subject.
Virtual reality (VR) technologies have also gained significant attention in the educational landscape. Wang and Hodgers (2021) [4] propose a cognitive VR classroom design, leveraging AI-enabled cognitive systems to create personalized and adaptive learning experiences. In this virtual environment, learners engage in realistic simulations, collaborative problem-solving tasks, and interactive scenarios that promote active learning. AI algorithms analyze learners’ responses and behaviors in real-time, adapting the learning content and environment to their individual needs and preferences. Such AI-enabled VR experiences offer unprecedented opportunities for experiential learning and enhanced cognitive development.
AI technologies can also assist students with diverse needs and skills. Educational platforms can offer adaptive learning paths, specialized recommendations, and personalized interventions based on individual student profiles using AI algorithms. Educators can address students’ unique strengths and weaknesses through this personalized approach, maximizing their learning potential (Kim et al., 2016) [2] . AI-enabled tools and platforms provide learners with adaptive assessments, real-time feedback, and targeted interventions that support their cognitive development.
5. Leveraging AI for Personalized and Optimized Learning Experiences
AIED has experienced remarkable advancements, driven by cutting-edge technologies such as artificial neural networks, machine learning, and Computer-Assisted Language Learning (CALL). These transformative technologies have enabled AIED to extend its reach beyond traditional classrooms, permeating diverse learning environments that include formal, non-formal, and informal settings (Holmes et al., 2019 [8] ; Adams et al., 2023 [7] ). As a result, AIED has revolutionized instructional methods, technological advancements, and educational research, redefining the way we approach teaching and learning.
By incorporating AIED, educators and learners are empowered to embrace computer-assisted collaborative learning and asynchronous discussion groups, promoting collaboration and active engagement. Moreover, AIED facilitates cost-effective personalized learning experiences through algorithm-driven navigation systems that adapt educational content to cater to individual needs and preferences. This personalized approach has shown significant potential in improving learning outcomes. Additionally, the integration of automated assessment, facial recognition systems, and predictive analytics within AIED enhances the evaluation process and enables personalized feedback (Adams et al., 2023) [7] .
A key advantage of AIED is its ability to leverage brain-based learning theories and provide personalized and optimized learning experiences for students. AI algorithms analyze vast amounts of data, including student performance, learning styles, preferences, and progress, to modify instructional strategies and offer personalized recommendations (Wang & Hodgers, 2021) [4] . This analysis enables AI to create individualized learning pathways tailored to each learner’s specific needs and skills, ultimately enhancing student outcomes.
AI and large language models (LLMs), an intelligent (AI) model that is designed to understand and generate human language, have spurred the development of various educational technology innovations aiming to automate time-consuming and laborious tasks such as generating and analyzing textual content (e.g., generating open-ended questions and analyzing student feedback surveys). LLMs, such as Bidirectional Encoder Representations from Transformers (BERT) and Generative Pre-trained Transformer (GPT), utilize deep learning and self-attention mechanisms to selectively attend to different parts of input texts, enabling the model to learn complex patterns and relationships among textual contents, such as their semantic, contextual, and syntactic relationships (Yan et al., 2023) [9] .
Several LLMs (e.g., GPT-3 and Codex) have been pre-trained on massive amounts of data across multiple disciplines, allowing them to perform natural language processing tasks with little (few-shot learning) or no additional training (zero-shot learning). This capability lowers the technological barriers for LLMs-based innovations, as researchers and practitioners can develop new educational technologies by fine-tuning LLMs on specific educational tasks without starting from scratch. The recent release of ChatGPT, an LLMs-based generative AI chatbot that requires only natural language prompts without additional model training or fine-tuning, has further reduced the barrier, making it accessible to individuals without a technological background to leverage the generative powers of LLMs (Yan et al., 2023) [9] .
Bada and Jita (2022) [1] research findings further support the notion that AI can personalize instruction and enhance the learning process. They highlight the cognitive VR classroom design proposed by Wang and Hodgers (2021) [4] , where AI algorithms observe and analyze students’ learning behaviors and responses within a virtual reality environment. Based on these observations, the AI system provides real-time feedback, suggestions, and adaptive interventions to guide students through their learning journey. For instance, if a student struggles with a specific concept, the AI system offers additional resources, alternative explanations, or interactive simulations customized to their unique needs. This individualized approach ensures that each student receives instruction aligned with their requirements, maximizing their learning potential.
Furthermore, AI systems with Machine Learning (ML) capabilities continuously adapt and refine their lesson plans based on ongoing student feedback and performance data. By identifying patterns, trends, and correlations in student data, AI guides instructional decision-making and improves the learning experience (Wang & Hodgers, 2021) [4] . Successful teaching strategies, content delivery techniques, and assessment methods are identified by the AI system, leading to continuous enhancement and optimization of the learning process.
These research findings emphasize that by leveraging AI to personalize and optimize learning experiences, educators can effectively enhance student engagement, motivation, and achievement. AI’s adaptive nature enables differentiated instruction, ensuring that students receive appropriate levels of challenge and support based on their individual progress and learning pace. As students engage in activities and receive feedback tailored to their unique strengths and areas for development, this personalized approach fosters self-directed learning, critical thinking, and problem-solving skills (Wang & Hodgers, 2021 [4] ; Bada & Jita, 2022 [1] ).
6. Assessing the Effectiveness of AI-Powered Brain-Based Learning Approaches
Numerous empirical studies have demonstrated the effectiveness of brain-based learning techniques powered by AI in improving various aspects of student learning. As shown in Table 1, Yilmaz and Yilmaz (2022) [10] examines student satisfaction with the use of an AI-powered learning system, specifically a smart Massive Open Online Course (MOOC) environment that incorporates various components such as learning analytics, recommendation systems, adaptive learning, adaptive mastering tests, dynamic assessment, and intelligent tutoring systems. The research was conducted with 51 students enrolled in the statistical methods in education course offered through the smart MOOC environment. Data was collected through a satisfaction questionnaire specifically developed for the smart MOOC environment.
The findings of the study indicate that the majority of students expressed
![]()
Table 1. Descriptive statistics on students’ general satisfaction with Adaptive-Dynamic-Intelligent Teaching System (ADITS).
Note. Adapted from Examining student satisfaction with the use of smart MOOC by Ramazan Yilmaz and Fatma Gizem Karaoğlan Yilmaz, 2022, 10th International İstanbul Scientific Research Congress.
satisfaction with the smart MOOC system and found it helpful for their learning. Students reported being able to access the information and resources they needed within the system. Additionally, students found the support services provided by the system to be useful, particularly in terms of exam preparation. They also expressed satisfaction with the instructional support, such as hints, similar examples, and guiding explanations, provided by the system when encountering difficulties while solving questions. Overall, the study suggests that students’ general satisfaction with the smart MOOC environment is high, which facilitates its acceptance and use (Yilmaz & Yilmaz, 2022) [10] .
The integration of adaptive learning and personalized features in the smart MOOC environment aligns with the need for individualized learning experiences that cater to students’ unique needs. The system utilizes data mining and machine learning techniques to analyze students’ behavior and provide personalized recommendations and guidance through a dashboard. This data-driven approach enables the system to create tailored learning pathways for each student, enhancing their learning outcomes.
Dewi and Kusumah (2019) [11] investigated the implementation of a brain-based learning approach using the internet to help students develop their mathematical reasoning abilities. The study showed that AI-enabled interventions improved students’ problem-solving skills and mathematical understanding. The AI algorithms used in the intervention provided individualized feedback and support, fostering the growth of students’ critical-thinking abilities and increasing their interest in the subject.
Tanner, Inoue, and Osterhout (2018) [12] explored individual differences in online second language (L2) sentence comprehension based on brain activity. The study aimed to enhance the accuracy and speed of learners’ comprehension through AI-based feedback mechanisms. The results demonstrated that AI-based feedback significantly improved learners’ comprehension skills, leading to greater accuracy and faster processing of L2 sentences. This highlights how AI can support language learners by providing individualized support and focused feedback based on their unique needs. These studies provide strong evidence that brain-based learning methods powered by AI can enhance student engagement, knowledge retention, and critical thinking abilities. The integration of AI enables the creation of individualized learning environments that cater to students’ learning preferences, styles, and areas for improvement. By utilizing AI technologies, educators can improve instructional methods and offer targeted support, leading to improved student learning outcomes.
7. Challenges and Ethical Considerations in AI-Enabled Brain-Based Learning
While incorporating AI into brain-based learning methodologies offers numerous advantages, it also presents challenges and ethical considerations that must be carefully addressed. Ethical implications are a significant area of concern in AI-enabled brain-based learning (McCall, 2020) [5] . For example, Key ethical issues include data privacy, algorithmic biases, and the responsible use of AI.
Data privacy is a critical concern when implementing AI in educational settings, as large-scale collection and analysis of student data raise questions about data storage, access, and usage. It is imperative to establish clear policies and procedures to safeguard the confidentiality and privacy of student information, ensuring the protection of personal data and upholding students’ rights and privacy (Adams et al., 2023) [7] .
However, in the reviewed studies, privacy concerns related to LLMs were rarely addressed. Specifically, there was a lack of explicit explanations regarding data collection consent and protection measures. This raises potential issues, as LLMs that work with stakeholders’ natural languages could inadvertently expose private information without proper informed consent (Yan et al., 2023) [9] . Addressing these privacy concerns is crucial to responsibly harness the power of LLMs in education while respecting individual privacy and data protection.
Algorithmic biases pose another ethical challenge. AI algorithms are trained using existing data, which may reflect societal prejudices and biases. Failure to acknowledge and address these biases can result in AI systems reinforcing stereotypes or perpetuating inequalities in educational settings. To mitigate the risk of bias and ensure fairness and equity in the learning process, careful assessment and monitoring of AI algorithms are necessary (Agarwal et al., 2023) [13] .
Moreover, caution should be exercised to avoid overreliance on AI in brain-based learning. While AI technologies can provide valuable insights and support, it is essential to maintain a balanced approach that recognizes the unique role of human educators. AI should supplement and enhance human interaction and guidance in the educational process, rather than replacing them. Striking a balance between retaining the human element and leveraging AI’s capabilities is crucial in using AI technologies in education.
To address these challenges and ethical concerns, educators and policymakers must establish clear guidelines and frameworks for the responsible integration of AI in brain-based learning. This includes fostering a culture of ethical AI use in educational institutions, implementing mechanisms to identify and mitigate algorithmic biases, and establishing transparent processes for data collection and usage.
By addressing these challenges and ethical considerations, educators can ensure that AI-enabled brain-based learning is implemented in a way that promotes equity, student autonomy, and responsible use of technology. This approach enhances students’ cognitive development while creating a morally upright and inclusive learning environment.
8. Practical Guidelines for Harnessing AI in Brain-Based Learning
In order to effectively harness the power of AI in brain-based learning, it is important for educators and policymakers to adhere to practical guidelines that consider essential factors such as data privacy, pedagogical integration, and ongoing professional development. These recommendations aim to empower educators to design innovative, engaging, and stimulating learning environments that promote cognitive development.
Data privacy emerges as a critical factor when integrating AI into brain-based learning. Safeguarding the security and privacy of student data is essential, as AI systems rely on extensive data to tailor instruction (Hairon & Chai, 2012) [14] . To protect sensitive student information, educators and policymakers must establish robust data protection measures, including encryption and secure storage.
Pedagogical integration is another significant consideration when utilizing AI in brain-based learning. Hairon and Chai (2012) [14] advocate for a shift from conventional pedagogical methods to becoming learning experience designers. This involves designing learning activities and environments that incorporate AI technologies to foster active participation, critical thinking, and problem-solving skills in learners. For instance, educators can use AI-powered virtual reality simulations to create immersive and interactive learning experiences that align with the principles of brain-based learning (Wang & Hodgers, 2021) [4] .
Continuous professional development is crucial for the successful integration of AI in brain-based learning. Educators need support and training to acquire the necessary skills and knowledge for effectively incorporating AI technologies into their teaching practices (Hairon & Chai, 2012) [14] . Access to professional development programs enables education professionals to explore best practices, learn about AI applications in the classroom, and collaborate with subject-matter experts.
Collaboration among educators, researchers, and technology developers is also essential for the successful integration of AI in brain-based learning. Through partnerships, useful AI tools and resources can be created in accordance with the principles of brain-based learning (Kim, Park, & Lee, 2016) [2] . Such collaborations enable educators to access the latest AI technologies and learn from research to inform their instructional practices.
By considering these practical guidelines, educators can effectively utilize AI in brain-based learning, transforming their roles from traditional pedagogues to creators of cutting-edge educational experiences. This integration of AI technology has the potential to enhance cognitive development and design stimulating learning environments.
9. Long-Term Implications of AI-Enabled Brain-Based Learning
AI-enabled brain-based learning has significant long-term implications for educational systems, workforce readiness, and lifelong learning opportunities. This innovative approach equips students with the necessary skills for the future workforce, transforming conventional educational practices. By leveraging AI technologies to personalize and optimize learning experiences, students can develop a deep understanding of complex ideas and enhance their critical thinking and problem-solving abilities.
Lifelong learning represents a significant long-term effect of AI-enabled brain-based learning. Unlike traditional education’s one-size-fits-all approach, AI empowers students through individualized, adaptive learning experiences tailored to their unique needs and preferences. AI algorithms provide personalized feedback, suggestions, and resources aligned with students’ learning styles and pace. This personalized approach not only enhances knowledge acquisition but also cultivates a lifelong love for learning, as students experience success and engagement throughout their educational journey.
Moreover, AI-enabled brain-based learning promotes the development of essential 21st-century skills required for success in a rapidly changing workplace. As AI technologies advance, the demand for individuals with higher-order cognitive abilities such as critical thinking, problem-solving, creativity, and adaptability increases. Integrating AI into brain-based learning allows educators to design learning experiences that actively engage students in real-world problem-solving tasks, collaborative projects, and creative exploration. This equips students with the knowledge and skills needed to navigate the challenges of the future workforce and evolving technology.
AI has the potential to transform educational systems by improving their effectiveness and efficiency. AI-powered tools can automate administrative tasks like grading and data analysis, freeing up more time for educators to focus on instructional design, individualized support, and personalized feedback. This shift enables educators to adopt a facilitative role, guiding students along their learning paths and providing targeted interventions when necessary. Additionally, AI algorithms can analyze extensive datasets to identify trends, patterns, and insights that inform curriculum development, instructional design, and decision-making in the field of education. While the long-term effects of AI-enabled brain-based learning are promising, it is crucial to address ethical issues and integration challenges. Responsible implementation requires careful consideration of privacy concerns, data security, algorithmic biases, and ethical use of learner data. Adequate training and professional development programs are also essential to ensure that educators are competent in utilizing AI technologies ethically and effectively in educational contexts.
10. Case Studies and Best Practices in AI-Enabled Brain-Based Learning
Insights into the potential of AI in enhancing cognitive development and revolutionizing education can be gained by examining case studies and best practices from educational institutions that have successfully incorporated AI and brain-based learning principles. One of such case study is provided by Khosravi et al. (2022) [15] , which presents FUMA, a framework designed to model and support students in exploratory, open-ended learning environments (OLE), such as interactive simulations, educational games, and MOOCs as shown in Figure 1. FUMA utilizes unsupervised clustering and association rule mining techniques on student interaction data within a specific Online Learning Environment. The primary objective of FUMA is to identify distinct classes of exploratory behaviors that can either facilitate or hinder learning outcomes. The framework then applies supervised machine learning to construct classifiers that can predict, in real-time, whether a student is effectively learning through exploratory interactions. In cases where effective learning is not occurring, FUMA identifies the specific behaviors responsible for this outcome. Subsequently, the system generates personalized hints to guide the student towards more effective utilization of the available tools and affordances within the OLE.
According to Wang and Hodgers (2021) [4] a noteworthy illustration is the conceptual learning design of the cognitive VR classroom. Their investigation into the cognitive VR classroom offers personalized and adaptive learning experiences by utilizing AI-enabled cognitive systems. AI algorithms assess students’ learning styles and offer real-time feedback, ensuring customized instruction and the best learning outcomes.
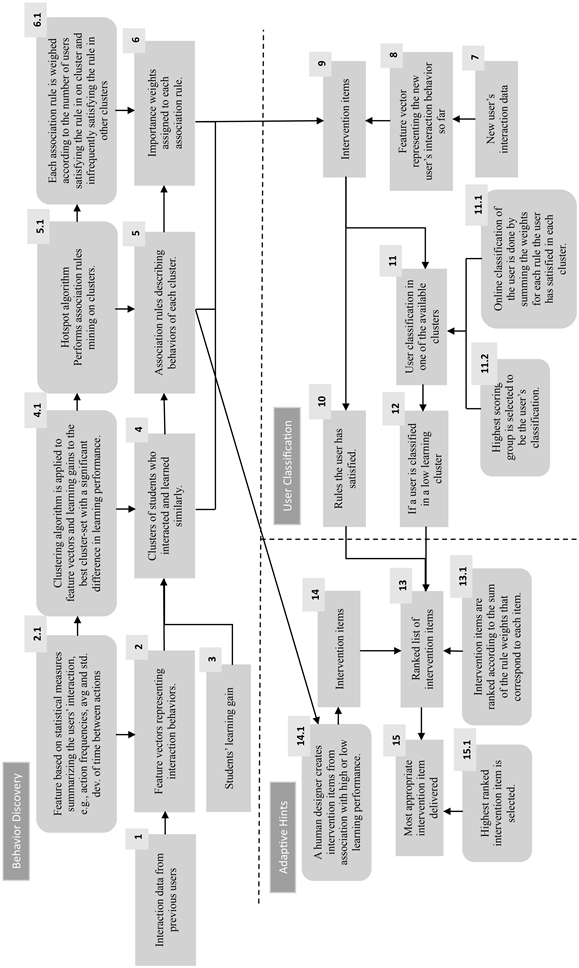
Note: Adapted from Explainable artificial intelligence in education, by Khosravi et al. 2022, Computers and Education: Artificial Intelligence.
Figure 1. User Modeling Framework broken down into three phases: Behavior Discovery, User Classification, and Adaptive Hints; rectangular nodes represent inputs and states, oval nodes represent processes.
These case studies highlight the importance of incorporating AI technologies into learning environments in order to strengthen brain-based learning strategies. They demonstrate how AI can be used to design immersive and interactive learning environments that are tailored to the needs and preferences of specific learners. Teachers can design adaptive learning pathways that maximize student engagement, motivation, and knowledge acquisition by utilizing AI’s capacity to analyze massive amounts of data and offer personalized recommendations.
Additionally, El-Wakeel’s (2020) [16] research on the application of brain-based learning techniques in the teaching of visual arts and design provides insights into real-world best practices. The study shows how incorporating brain-based learning concepts into these disciplines promotes creativity, critical thinking, and problem-solving abilities. Teachers can enhance these learning opportunities by incorporating AI technologies, giving students the opportunity to explore and develop their artistic abilities while receiving individualized coaching and feedback.
These case studies and best practices demonstrate how AI-enabled brain-based learning has the potential to revolutionize numerous academic fields. They show how incorporating AI technologies, such as virtual reality and interactive displays, can foster improved cognitive development and transform conventional educational approaches. By utilizing AI, educators can develop cutting-edge and engaging learning environments that support active learning, individualized instruction, and the best possible student outcomes.
11. Strategies for Scaling and Implementing AI-Based Brain-Based Learning
Infrastructure, training, and collaboration are crucial considerations when implementing AI-based brain-based learning approaches. Educators must ensure that the necessary technological infrastructure, such as a reliable internet connection and access to devices like computers or tablets, is in place to support the integration of AI technologies in the classroom (Kim, Park, & Lee, 2016) [2] .
In addition to infrastructure, educators and instructional staff require proper training to effectively utilize AI technologies and incorporate brain-based learning strategies into their pedagogical practices. Professional development programs play a key role in equipping teachers with the knowledge and skills needed to effectively use AI tools and techniques (McCall, 2020) [5] . Ongoing training and support are essential to ensure educators can navigate the complexities of AI-enabled brain-based learning. Successful implementation of AI-based brain-based learning approaches also relys on collaboration between educators, researchers, and technology developers.
By working together, these stakeholders can address the unique opportunities and challenges presented by AI in education, exchange knowledge, share best practices, and create innovative solutions (Hairon & Chai, 2012) [14] . Collaborative efforts can lead to the development of AI tools tailored to the needs of learners and the creation of efficient instructional strategies.
El-Wakeel (2020) [16] highlights the importance of incorporating brain-based learning techniques in the teaching of visual arts and design. This integration can offer valuable insights into effective tactics for implementing AI-enabled brain-based learning techniques. Encouraging creativity and incorporating experiential, hands-on learning activities can increase student engagement and foster a deeper understanding of the material (Creating Minds, 2020).
To effectively scale and implement AI-based brain-based learning approaches, educators and policymakers must consider aspects such as infrastructure, training, and collaboration. These calculated efforts are necessary to realize the transformative potential of AI in improving cognitive development and transforming education.
The synergistic relationship between brain-based learning and AI has the potential to significantly enhance cognitive development and revolutionize education. Brain-based learning, grounded in an understanding of how the brain processes information and learns, provides a theoretical framework for designing effective instructional strategies (Ramakrishnan, 2018) [3] . AI technologies, with advanced data analysis and machine learning capabilities, enable the personalization and enhancement of learning experiences (Wang & Hodgers, 2021) [4] .
Integrating AI into brain-based learning allows educators to design individualized and adaptive learning environments that cater to each student’s unique needs and preferences. AI algorithms can analyze vast amounts of data, including learner performance, preferences, and cognitive patterns, to deliver personalized instruction and interventions, optimizing the learning process (Dewi & Kusumah, 2019) [11] .
Real-time adaptability is a significant advantage of AI-enabled brain-based learning. AI systems can monitor students’ progress, identify areas of strength or weakness, and provide immediate feedback and guidance. This real-time adaptability ensures that students receive timely support, enhancing their motivation and engagement (Tanner, Inoue, & Osterhout, 2018) [12] .
AI technologies also facilitate the incorporation of multimodal and immersive learning activities known to increase engagement and knowledge retention (Kim, Park, & Lee, 2016) [2] . For example, combining virtual reality (VR) environments with AI algorithms enables the creation of immersive and interactive learning scenarios that simulate real-world experiences (Wang & Hodgers, 2021) [4] . Research has shown that these experiences enhance learning and deepen understanding.
AI’s ability to identify patterns and trends in learning data empowers educators to make informed decisions regarding curriculum development, instructional methods, and educational policies (El-Wakeel, 2020) [16] . By leveraging AI technologies, educators gain valuable insights into student progress, areas of strength, and areas that require additional support, allowing them to adjust their lesson plans accordingly.
While the synergistic relationship between brain-based learning and AI holds tremendous potential, certain considerations must be kept in mind. McCall (2020) [5] highlights concerns about data collection, algorithmic biases, and the responsible application of AI. Transparent and ethical practices in data collection and analysis, along with ongoing monitoring and evaluation, are essential to maintain trust and ensure equity in educational settings where AI is used.
12. Discussion
To foster cognitive growth and improve learning experiences, the integration of AI-enabled brain-based learning has the potential to revolutionize education. This section discusses the implications and advantages of brain-based learning supported by AI as well as the difficulties and factors that need to be taken into account.
12.1. Implications and Benefits of AI-Enabled Brain-Based Learning
1) Personalized and Adaptive Instruction: AI technologies can examine a large amount of information about a particular learner, such as their preferences, learning styles, and level of development. AI-enabled systems can deliver personalized and adaptive instruction catered to each learner’s particular needs and abilities by making use of this data. As students receive instruction that is tailored to their individual needs, this individualized approach encourages self-directed learning, engagement, and motivation (Wang & Hodgers, 2021) [4] .
2) Cognitive Development: By combining brain-based learning principles with AI technologies, the learning process can be optimized, and cognitive development can be improved. Brain-based learning is in line with how the brain naturally processes information by involving multiple senses, encouraging active learning, and offering opportunities for reflection and application. By offering immersive experiences, real-time feedback, and adaptive interventions that deepen understanding and encourage long-term knowledge retention, AI technologies further improve these processes (Ramakrishnan, 2018 [3] ; Wang & Hodgers, 2021 [4] ).
3) Accessible and Inclusive Education: AI-enabled brain-based learning can support students with a range of needs and abilities, encouraging inclusivity in the classroom. AI technologies can address the strengths, weaknesses, and learning pace of individual learners through adaptive learning paths, individualized interventions, and tailored recommendations. Regardless of their backgrounds or skills, all students will receive the appropriate level of support and challenge thanks to this personalized approach (Kim et al., 2016) [2] .
12.2. Challenges and Considerations
1) Ethical Use of Student Data: In AI-enabled brain-based learning, ethical and responsible use of student data is essential. In order to safeguard student confidentiality and adhere to legal and moral requirements, educational organizations and personnel must place a high priority on data privacy and security. Building trust and ensuring the responsible use of student data requires transparency, informed consent, and strong data protection measures (McCall, 2020 [5] ; Yan et al., 2023 [9] ).
2) Algorithmic Biases: Artificial intelligence (AI) algorithms are trained using historical data, which may be biased and unequal. These biases have the potential to persist in AI-enabled educational systems and produce unfair results if they are not carefully observed and addressed. To find and reduce algorithmic biases and ensure fairness and equity in learning environments utilizing AI, rigorous evaluation and validation processes are required (Wang & Hodgers, 2021) [4] .
3) Striking a Balance: Balancing the roles of AI and human involvement in education is essential, even though AI technologies provide insightful information and support. The human element, which includes teachers’ knowledge, compassion, and social-emotional support, continues to be crucial for promoting deep connections and comprehensive education. The role of educators as mentors and facilitators should be enhanced by AI rather than replaced by it (Hairon & Chai, 2018) [14] .
12.3. Future Directions and Research Implications
The investigation of brain-based learning supported by AI opens doors for additional study and development. Future research could explore how AI-enabled interventions affect academic achievement, knowledge retention, and cognitive development over the long term. In order to address concerns about algorithmic biases and ensure fairness in educational settings, research might also concentrate on designing AI algorithms that are more open, understandable, and accountable. Additionally, research into the function of educators in environments with AI capabilities and the creation of training programs to give teachers the knowledge and skills they need to successfully incorporate AI into instruction would be beneficial.
13. Conclusions
The exploration of the potential of AI-enabled brain-based learning for improved cognitive development is driving a revolution in education that has the potential to fundamentally alter teaching and learning methods. This study delved into brain-based learning theories and explored the current state of AI education technology, focusing on the potential synergy of Artificial Intelligence in Education (AIED), Machine Learning (ML), and Large Language Model (LLM) applications. The findings underscored the positive impact of brain-based learning practices, such as creating encouraging environments, incorporating multisensory experiences, promoting active learning, and encouraging reflection and application, all of which influence students’ motivation, engagement, and knowledge retention. The integration of AI technologies in education opens new possibilities for enhancing these principles and adapting instruction to cater to individual students’ specific needs.
Innovative AI applications in education are already emerging, with cognitive VR classroom designs and museum learning models grounded in brain-based learning principles. These AI-enabled systems offer interactive and immersive learning environments, fostering personalized and flexible learning experiences. Leveraging AI algorithms for real-time feedback, suggestions, and interventions contributes to the personalization and improvement of the learning process.
By harnessing AI to personalize and optimize learning experiences, educators can effectively meet the diverse needs and abilities of students. AI-based systems’ adaptability allows for differentiated instruction, ensuring that each student receives appropriate challenges and support, fostering independent learning, critical thinking, and problem-solving abilities, ultimately enhancing cognitive growth and academic success.
While the convergence of brain-based learning and AI holds immense promise, it is vital to address the associated challenges and ethical issues. Factors such as data privacy, algorithmic biases, and equitable access to AI technologies require careful consideration to create a fair and inclusive learning environment.
In conclusion, the fusion of brain-based learning with AI has the potential to revolutionize education. Embracing AI-enabled brain-based learning approaches empower educators and decision-makers to design optimal learning environments, enhancing cognitive development, student engagement, and knowledge retention. The practical guidelines derived from this research offer valuable insights for effectively leveraging AI in education. Educators and policymakers must stay informed, adapt their practices, and explore new ways to harness the potential of AI-enabled brain-based learning for the benefit of students and the future of education as AI technologies continue to advance and evolve.