Understanding Model Independent Genetic Mutations through Trends in Increase in Entropy ()
1. Introduction
Genetic entropy can be defined as the genetic breakdown of living things. As biological processes and mishaps occur, genetic entropy increases while the relationships between living things in the body become increasingly more chaotic and complex. Mutations occur when the body codes for the wrong gene in a DNA sequence, whether it be from the environmental factors such as UV Radiation, or intrinsic factors such as errors in DNA synthesis and replication. Genetic entropy increases when things become less ordered, while mutations are humanity’s physical manifestation of less order, in that they cause an increase in complexity every time they occur. As a result, it seems clear that genetic mutations increase genetic entropy by increasing amounts of disorder. There seem to be two primary ways by which genetic mutations affect the genetic entropy of humans. The first effect of mutations on genetic entropy occurs at an individual level. This process of entropy, also called biosemiotic entropy, describes an “error or deviation from a healthy state.” Crucially, cancer is an accurate representation of biosemiotic entropy. Error in genetic code builds onto more errors, as the corrosion of code causes deletions and insertions to become huge frameshifts, and the accumulation of these random mutations ultimately causes an error in the cell-cycle control mechanism. When the cell loses control over its control mechanisms, a cancerous tumor develops while blood and nutrients are drawn toward the growth. The biosemiotic entropy of humans increases over the course of people’s lives, so by the end of a human’s life, the cells have accumulated thousands of mutations, which ultimately causes problems like cancer. The second effect of mutations on entropy occurs on an evolutionary basis more so than it does on a population-wide basis. As researchers have made clear, genetic mutations can not be spread through populations of people, one person cannot give their neighbor Duchenne’s Syndrome or Red-Green Color-Blindness. However, evolution dictates that mutations, can be passed on from one generation to the next. We know that mutations stack up over time in an individual, and genetic entropy thereby increases. As a result, a population over time will constantly increase its genetic entropy as more disorder occurs in a genome. Overall, it is clear that genetic entropy is constantly increasing in the world, as chaos causes more chaos.
In biology, mutations are defined as alterations to the sequence of nucleotides in genetic material, whether it be DNA, RNA, proteins, or cells [1] [2] [3]. UV and ionizing radiation, chemical mutagens, viruses (such as lentiviruses and adenovirus as used in vectors), copying errors, and hyper-mutation are just some of the methods by which mutations may occur.
In eukaryotic organisms with germ cells, or reproductive cells, there are two types of common mutations. The first type, germ line mutations, can be transmitted to offspring via reproductive cells. Alternatively somatic mutations, which involve somatic or body cells, can not be transmitted to offspring [3] [4].
Asexual organisms often have different mechanisms of reproduction such as cuttings or budding, which can make the distinction between mutations become confusing. For example, some plants transmit somatic mutations in somatically mutated parts of plants where flower buds develop, which can be considered both asexual and sexual. Another mutation type is a de novo mutation, in which genetic mutations arise from DNA sequences that were not always meant to be coded or undergo transcription and translation. While the source of these mutations is unrelated to the effects of the mutation, the type of cell may play a role in whether negative impacts occur.
Finally, in general, mutation is widely considered the mechanism upon which natural selection acts, causing advantageous genetic material and traits to multiply through survival, while causing disadvantageous genetic material and traits to reduce in quantity by dying out. As mentioned previously, the last important piece of information we mention in this paper is that mutations can be the result of inserted or deleted DNA through plasmids and vectors, different types of mobile genetic elements [5] [6] [7].
In this article we will study the statistical physics of model independent mutations. We will show that the entropy of a successful mutation and a failed mutation is different from the entropic aspects and the range in which the entropy of both types of mutations lie.
2. Organization of This Paper
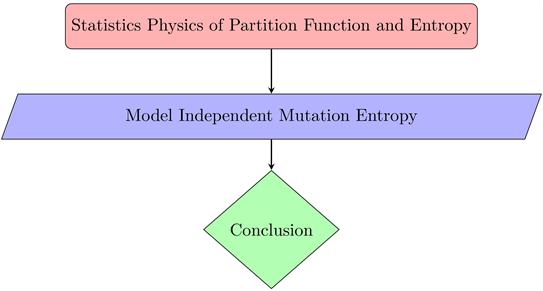
3. Statistics Physics of Partition Function and Entropy
3.1. Partition Function
Partition functions describe the statistical properties of a system and are defined by functions of the thermodynamic state variables, such as the temperature and volume. Most of the thermodynamic variables of the system, such as the total energy, free energy, entropy, and pressure, can be expressed in terms of the partition function. Every partition function can be constructed in a way so that it represents a statistical ensemble.
The partition function can be defined as:
(3.1)
where
,
is the Boltzmann constant and T is the temperature.
3.2. Average Energy
The average energy or the expectation value of the energy is given as:
(3.2)
Using Equation (3.1) we get
(3.3)
3.3. Variance in Energy
The variance in the energy of a system or the fluctuation in energy can be calculated by:
(3.4)
3.4. Entropy
The entropy is a thermodynamic quantity which is defined as the chaos in a system. It can be expressed with relation to the partition function as:
(3.5)
4. Model Independent Mutation Entropy
In this section we have used the temperatures and the average energies of a normal mutation as shown in Figure 1, and that of a failed mutation as shown in Figure 2. The energies have been scaled down to clearly express the ranges of entropies. The figures clearly indicate that the entropy range in a failed mutation is much higher compared to the entropy range in a normal mutation. The energies and the temperatures have been cited from [8] [9] [10] [11]. There are more
![]()
Figure 1. The entropy trends in a normal mutation.
![]()
Figure 2. The entropy trends in a failed mutation.
studies which show detailed calculations of energies of very specific mutations [12] [13] [14] [15] [16]. We have limited our study to a general model independent approach. The graphs below show the plots of entropy vs energy in the cases of a normal mutation and a failed mutation.
In both figures, the blue lines indicate the lower limit of the entropy increase and the red line indicates the upper limit of the entropy increase.
There are other ways to measure the entropy of a biosystem using Fokker-Planck equation which is discussed in detail in [17] - [22]. In these articles, the entropy is calculated in detail of a complex non-equilibrium biosystem.
5. Conclusion
In this study we have made an attempt to understand model independent mutations of normal and failed types through basic thermodynamics. We have shown that the entropy change for a normal mutation is much less compared to the entropy change in a failed mutation. This study is a very general one and not specific to any particular type of mutation. However, the figures show that if we apply this study to any specific mutation, the entropies should lie within the range as shown in the plots or close to the range within reasonable uncertainties. Our future work is to study a specific type of mutation and see how the basics of statistical and thermodynamical physics explain the mutations from an entropy understanding.
Acknowledgements
The authors thank Paolo Grigolini, Randal Hallford, Yelena Nemchen and Garrett Baughman for useful discussions throughout the project.